A beginner’s guide to the AI apocalypse: Killer robots
Welcome to the fifth article in TNW’s guide to the AI apocalypse. In this series we examine some of the most popular doomsday scenarios prognosticated by modern AI experts. Previous articles in this series include: Misaligned Objectives , Artificial Stupidity , Wall-E Syndrome , and Humanity Joins the Hivemind .
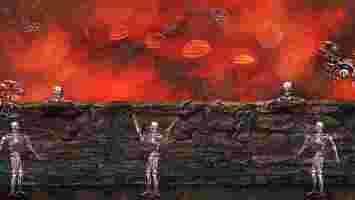
We’ve danced around the subject of killer robots in the previous four editions in this series, but it’s time to look the machines in their beady red eyes and… speculate.
First things first: the reason why we haven’t covered ‘killer robots‘ in this series so far is because it’s an incredibly unlikely doomsday scenario. The seminal film about killer robots, Terminator 2: Judgment Day, paints the vivid picture of a global AI takeover involving armies of killer robots hunting humans down like vermin and eradicating them on sight.
But, even in today’s modern age of AI-everything, that particular scenario remains highly unlikely. Mostly because it’s hard to imagine a rational, sentient AI would see violence as a viable solution to any problem. The gist of the argument here is that killing humans doesn’t benefit AI. And killing robots shouldn’t bother AI because we can assume any strong AI will have access to the cloud.
In the words of GPT-3 , an AI-powered text generator:
[
Okay, perhaps the robot doth protest too much, the “everything in my power to fend off any attempts at destruction” part seems a bit gratuitous. But it does intimate the real problem: if killer robots ever come after humanity it probably won’t be at the order of an AI. It’ll almost surely be humans that sick robots on other humans.
So if we don’t have to worry about armies of giant killer robots marching through the streets using heat sensors and lasers to destroy the last few remaining humans under the direction of an AI overlord, then why not imagine the same scenario with humans in charge?
Instead of Skynet (a fictional, sentient AI from the Terminator franchise) becoming self-aware and determining all humans must die, what if it was just a nasty dictator or evil CEO pulling the strings? The problem here is that, while giant robot armies full of ugly metal monstrosities and androids capable of passing as humans make for an awesome spectacle, they’re incredibly impractical.
Why would any self-serving evil government or super villain build giant robots when, for the cost of materials and energy it would take to build one Terminator unit, they could probably build thousands of tiny slaughterbots :
The point is, killing humans is far too simple a task to require giant mechanized monsters. Tiny balls full of sensors and a noxious substance, like a virus, for example, would do the trick much more efficiently than bipedal assassin bots.
Our bigger concerns, when it comes to killer robots, would be accidental death and dismemberment. Maybe your future robot butler suffers a brief software glitch with its touch and pressure sensors and accidentally rips your head off instead of fixing your necktie. Maybe a construction-bot has a malfunctioning physics sensor and starts building 20-story death traps instead of office buildings. Those types of situations could be problematic in the future.
But entire armies destroying precious resources the machines will need after we’re all gone? That sounds counterproductive. There’s very little chance AI will suffer our same self-destructive proclivities. Wars are almost always fought for selfish, ideological purposes.
So the bottom line is: We don’t need to stock up on tanks to fight the machines, we need to develop policies that stop humans from using them to harm other humans. If killer robots ever become a problem for humanity it’ll be our own fault.
How to use pre-trained models in your next business project
Most of the new deep learning models being released, especially in NLP , are very, very large: They have parameters ranging from hundreds of millions to tens of billions .
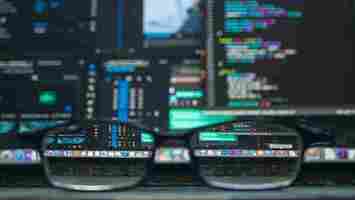
Given good enough architecture, the larger the model , the more learning capacity it has. Thus, these new models have huge learning capacity and are trained on very, very large datasets .
Because of that, they learn the entire distribution of the datasets they are trained on. One can say that they encode compressed knowledge of these datasets. This allows these models to be used for very interesting applications—the most common one being transfer learning . Transfer learning is fine-tuning pre-trained models on custom datasets/tasks , which requires far less data, and models converge very quickly compared to training from scratch.
How pre-trained models are the algorithms of the future
Although pre-trained models are also used in computer vision, this article will focus on their cutting-edge use in the natural language processing (NLP) domain. Transformer architecture is the most common and most powerful architecture that is being used in these models.
Although BERT started the NLP transfer learning revolution, we will explore GPT-2 and T5 models. These models are pre-trained—fine-tuning them on specific applications will result in much better evaluation metrics, but we will be using them out of the box, i with no fine-tuning.
Pre-trained NLP models: OpenAI’s GPT-2
GPT-2 created quite a controversy when it was released back in 2019. Since it was very good at generating text , it attracted quite the media attention and raised a lot of questions regarding the future of AI.
Trained on 40 GB of textual data, GPT-2 is a very large model containing a massive amount of compressed knowledge from a cross-section of the internet.
GPT-2 has a lot of potential use cases . It can be used to predict the probability of a sentence. This, in turn, can be used for text autocorrection. Next, word prediction can be directly used to build an autocomplete component for an IDE (like Visual Studio Code or PyCharm) for writing code as well as general text writing . We will use it for automatic text generation, and a large corpus of text can be used for natural language analysis.
The ability of a pre-trained model like GPT-2 to generate coherent text is very impressive. We can give it a prefix text and ask it to generate the next word, phrase, or sentence.
An example use case is generating a product reviews dataset to see which type of words are generally used in positive reviews versus negative reviews.
Let’s look at some examples, starting with what we get if we start with the positive prefix, “Really liked this movie!”
Sample 1 | Loved the character’s emotions at being in constant danger, and how his inner fears were slowly overcome by these events. Also loved that he is so focused on surviving; even for a while it felt like something out of Batman v Superman was showing up every now again because you always knew your enemy would show up eventually anyways :) The ending theme really stuck with me too… I mean yeah they did have to… |
Sample 2 | It did exactly what I wanted it to do, just a lot of fun. The cast was awesome and the story makes sense so far after seeing everything else already on IMDB. There are hints about being in an underground city from time immemorial due their “power” (or lack thereof) but they don’t really tell that much…I think its more like one big mystery then some other aspects coming together with me for now though….It’s supposed… |
As you can see, the word review was not anywhere in the prefix, but as most reviews are titles followed by the body of the review, this forced the model to adapt to that distribution. Also notice the reference to Batman v Superman .
Let’s see another example. Instead of a movie review, we’ll try to generate a product review using the negative prefix, “A trash product! Do not buy.”
Sample 1 | And please, do NOT try to use this as a fertilizer for your gardens or lawns – it will cause some serious water damage if ingested by fish and birds. Use with caution because of the possibility that pesticides may be present in pet foods available online at organic grocery stores such too “good” names like Petsmart, PetSmart & Safeway.How toxic is food waste? Waste products can contribute significant amounts – enough so that even very… |
Sample 2 | No one will give you a piece of that garbage ever again. Just do yourself and your business a favor and clean up before buying it for nothing more than to save some money or convenience in the future.1/2 oz Bottle Plastic Food Storage Packaging + 1 pack #1636370105702615 (P, 3ml) $7 Buy Now You can purchase these bottles at any retail stores but here is how to get… |
Again, the prefix can be inferred as the title of a product review, so the model starts generating text following that pattern. GPT-2 can generate any type of text like this.
A Google Colab notebook is ready to be used for experiments, as is the “Write With Transformer” live demo .
Yes, since GPT-2 is trained on the web, it “knows” a lot of human knowledge that has been published online up till 2019. It can work for contextual questions as well, but we will have to follow the explicit format of “Question: X, Answer:” before letting it attempt to autocomplete. But if we force the model to answer our question, it may output a pretty vague answer. Here’s what happens trying to force it to answer open-ended questions to test its knowledge:
Sample 1 | Question: Who invented the theory of evolution? Answer: The theory of evolution was first proposed by Charles Darwin in 1859. |
Sample 2 | Question: How many teeth do humans have? Answer: Humans have 21 teeth. |
As we can see, the pre-trained model gave a pretty detailed answer to the first question. For the second, it tried its best, but it does not compare with Google Search.
It’s clear that GPT-2 has huge potential. Fine-tuning it, it can be used for the above-mentioned examples with much higher accuracy. But even the pre-trained GPT-2 we are evaluating is still not that bad.
Google’s T5 is one of the most advanced natural language models to date. It builds on top of previous work on Transformer models in general. Unlike BERT, which had only encoder blocks, and GPT-2, which had only decoder blocks, T5 uses both .
GPT-2 being trained on 40 GB of text data was already impressive, but T5 was trained on a 7 TB dataset . Even though it was trained for a very, very large number of iterations, it could not go through all the text. Although T5 can do text generation like GPT-2, we will use it for more interesting business use cases.
Let’s start with a simple task: text summarization . For those AI development companies wanting to build an app that summarizes a news article, T5 is perfectly suited for the task. For example, giving this article to T5, here are three different summaries it produced:
V1 | destiny 2’s next season, starting march 10, will rework swords . they’ll have recharging energy used to power both heavy attacks and guarding . the valentine’s day event, crimson days, is also happening this month . |
V2 | bungie has revealed that the next season of destiny 2 will dramatically rework swords . the studio has mostly been coy about what the season will entail . the rethink will let swords partly bypass ai enemies’ shields . |
V3 | destiny 2’s next season will rework swords and let them bypass ai enemies’ shields . the season starts march 10th . you can play destiny 2 during crimson days, a valentine’s day event . |
As we can see, it has done a pretty nifty job of summarizing the article. Also, each summary is different from the others.
Summarizing using pre-trained models has huge potential applications. One interesting use case could be to generate a summary of every article automatically and put that at the start for readers who just want a synopsis. It could be taken further by personalizing the summary for each user . For example, if some users have smaller vocabularies, they could be served a summary with less complicated word choices. This is a very simple example, yet it demonstrates the power of this model.
Another interesting use case could be to use such summaries in the SEO of a website. Although T5 can be trained to generate very high-quality SEO automatically, using a summary might help out of the box, without retraining the model.
T5 can also be used for reading comprehension , e.g., answering questions from a given context. This application has very interesting use cases we will see later. But let’s start with a few examples:
Question | Who invented the theory of evolution? |
Context (Encyclopædia Britannica) | The discovery of fossil bones from large extinct mammals in Argentina and the observation of numerous species of finches in the Galapagos Islands were among the events credited with stimulating Darwin’s interest in how species originate. In 1859 he published On the Origin of Species by Means of Natural Selection, a treatise establishing the theory of evolution and, most important, the role of natural selection in determining its course. |
Answer | darwin |
There is no explicit mention that Darwin invented the theory, but the model used its existing knowledge along with some context to reach the right conclusion.
How about a very small context?
Question | Where did we go? |
Context | On my birthday, we decided to visit the northern areas of Pakistan. It was really fun. |
Answer | northern areas of pakistan |
Okay, that was pretty easy. How about a philosophical question?
Question | What is the meaning of life? |
Context (Wikipedia) | The meaning of life as we perceive it is derived from philosophical and religious contemplation of, and scientific inquiries about existence, social ties, consciousness, and happiness. Many other issues are also involved, such as symbolic meaning, ontology, value, purpose, ethics, good and evil, free will, the existence of one or multiple gods, conceptions of God, the soul, and the afterlife. Scientific contributions focus primarily on describing related empirical facts about the universe, exploring the context and parameters concerning the “how” of life. |
Answer | philosophical and religious contemplation of, and scientific inquiries about existence, social ties, consciousness, and happiness |
Although we know the answer to this question is very complicated, T5 tried to come up with a very close, yet sensible answer. Kudos!
Let us take it further. Let’s ask a few questions using the previously mentioned Engadget article as the context.
Question | What is this about? |
Answer | destiny 2 will dramatically rework |
Question | When can we expect this update? |
Answer | march 10th |
As you can see, the contextual question answering of T5 is very good. One business use case could be to build a contextual chatbot for websites that answers queries relevant to the current page.
Another use case could be to search for some information from documents, e.g., ask questions like, “Is it a breach of contract to use a company laptop for a personal project?” using a legal document as context. Although T5 has its limits, it is pretty well-suited for this type of task.
Readers may wonder, Why not use specialized models for each task? It’s a good point: The accuracy would be much higher and the deployment cost of specialized models would be much lower than T5’s pre-trained NLP model. But the beauty of T5 is precisely that it is “one model to rule them all,” i you can use one pre-trained model for almost any NLP task . Plus, we want to use these models out of the box, without retraining or fine-tuning. So for developers creating an app that summarizes different articles, as well as an app that does contextual question answering, the same T5 model can do both of them.
Pre-trained models: the deep learning models that will soon be ubiquitous
In this article, we explored pre-trained models and how to use them out of the box for different business use cases. Just like a classical sorting algorithm is used almost everywhere for sorting problems, these pre-trained models will be used as standard algorithms. It’s pretty clear that what we explored was just scratching the surface of NLP applications, and there is a lot more that can be done by these models.
Pre-trained deep learning models like StyleGAN-2 and DeepLabv3 can power, in a similar fashion, applications of computer vision .
The Toptal Engineering Blog is a hub for in-depth development tutorials and new technology announcements created by professional software engineers in the Toptal network. You can read the original piece written by Nauman Mustafa here . Follow the Toptal Design Blog on Twitter and LinkedIn .
US woman arrested after making Deepfakes of daughter’s cheerleading rivals
A Pennsylvania woman was arrested last week after police discovered she’d created “Deepfake” videos of her daughter’s cheerleading rivals in an apparent effort to harass and intimidate them.
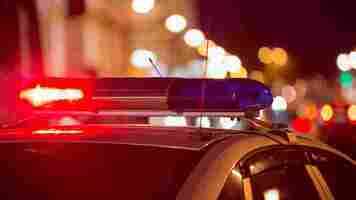
Charged with multiple misdemeanors, the woman’s alleged crimes involve sending videos to the cheerleading team’s coaches and the girls targeted that had been altered using AI-powered software to make it appear as though members of the team were engaging in lewd or rule-breaking behavior.
The case is being handled by Bucks County District Attorney Matt Weintraub’s office, which recently told reporters the woman also sent anonymous messages to the victims harassing them, including making statements urging the cheerleaders to take their own lives.
Local news reports indicate the woman’s actions were intended to force her daughter’s rivals off the squad – all indications are that the daughter was unaware of her mother’s actions.
Background: Detectives tracked down the woman after tracing phone numbers used to send messages to the cheerleaders. According to reports , the phone numbers lead to a company that sells Deepfakes to marketing teams. It’s unclear at this time if the woman works for the company, solicited its work, or created the Deepfakes herself.
Quick take: Don’t believe everything you see. Deepfakes have been around for a few years now, and a number of people have been arrested for their misuse.
We’ve seen them used to entertain and amaze , but it’s been clear since their inception that Deepfakes represent a danger to society.
Luckily, there’s still ways to detect Deepfakes . But that could change quickly as developers figure out how to overcome the platform’s shortcomings.
As the skill and technology-level required to discern the difference between a Deepfake and the real thing continue to rise, we’re almost certain to see more situations like this.
Greetings Humanoids! Did you know we have a newsletter all about AI? You can subscribe to it right here .