Building a virtual Earth could be the key for clean energy
More than 100 countries, including the United States and members of the European Union, have committed to net-zero carbon emissions by 2050. The world is going to need a lot of metal, particularly copper.
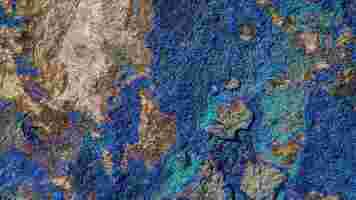
Recently, the International Energy Agency sounded the warning bell on the global supply of copper as the most widely used metal in renewable energy technologies. With Goldman Sachs predicting copper demand to grow up to 600% by 2030 and global supply becoming increasingly strained, it is clear we need to find new and large deposits of copper fast.
Getting this much copper will be impossible unless we discover significant new copper deposits. But there has been little exploration for copper over the past decade, as prices have been relatively low.
We have been developing software to model Earth in four dimensions to look deep inside the planet and back into the past to discover where copper deposits formed along ancient mountain ranges. This software, called GPlates , is a powerful four-dimensional information system for geologists.
How large copper deposits form
Many of the world’s richest copper deposits formed along volcanic mountain chains such as the Andes and the Rocky Mountains. In these regions, an oceanic tectonic plate and a continent collide, with the oceanic plate sinking under the edge of the continent in a process called subduction.
This process creates a variety of igneous rocks and copper deposits to form along the edge of the continent, at depths of between one and five kilometers in the crust, where hot magmatic fluids containing copper (and other elements) circulate within networks of faults. After millions of years of further plate movement and erosion, these treasures move close to the surface – ready to be discovered.
Searching for copper
Geologists typically use a set of well-established tools to look for copper. These include geological mapping, geochemical sampling, geophysical surveys, and remote sensing. However, this approach does not consider the origin of the magmatic fluids in space and time as the driver of copper formation.
We know these magmatic fluids come from the “mantle wedge”, a wedge-shaped piece of the mantle between the two plates that are fed by oceanic fluids escaping from the downgoing plate. The oceanic plate heats up on its way down, releasing fluids that rise into the overlying continental crust, which in turn drives volcanic activity at the surface and the accumulation of metals such as copper.
Differences in how subduction occurs and the characteristics of the oceanic plate may hold the secret to better understanding where and when copper deposits form. However, this information is traditionally not used in copper exploration.
Building a virtual Earth
At the EarthByte research group, we are building a virtual Earth powered by our GPlates plate tectonic software, which lets us look deep below the surface and travel back in time. One of its many applications is to understand where copper deposits have formed along mountain belts.
In a recent paper , we outline how it works. We focus on the past 80 million years because most of the known economic copper deposits along mountain belts formed during this period. This period is also most accurate for our models .
We used machine learning to find links between known copper deposits along mountain belts and the evolution of the associated subduction zone. Our model looks at several different subduction zone parameters and determines how important each one is in terms of association with known ore deposits.
So what turns out to be important? How fast the plates are moving towards each other, how much calcium carbonate is contained in the subducting crust and in deep-sea sediments, how old and thick the subducting plate is, and how far it is to the nearest edge of a subduction zone.
Using our machine learning approach, we can look at different parts of the world and see whether they would have experienced conditions conducive to forming copper deposits at different times. We identified several candidate regions in the US, including in central Alaska, southern Nevada, southern California, and Arizona, and numerous regions in Mexico, Chile, Peru, and Ecuador.
Knowing when copper ore deposits may have formed is important, as it helps explorers to focus their efforts on rocks of particular ages. In addition, it reveals how much time given deposits might have had to move closer to the surface.
Australia has similar deposits, including the Cadia copper-gold district in New South Wales. However, these rocks are significantly older (roughly 460 million to 430 million years old) and require virtual Earth models to look much further back in time than those applied to the Americas.
Future of mineral exploration
Finding 10 million tonnes of copper by 2030 – the equivalent of eight of the largest copper deposits that we mine today – presents an enormous challenge.
With the support of over a decade from AuScope and the National Collaborative Research Infrastructure Strategy (NCRIS) , we are in a position to imagine tackling this challenge. By supercharging GPlates in Australia’s Downward Looking Telescope , together with AI and supercomputing, we can meet it head on.
These emerging technologies are increasingly being used by Australian startups like Lithodat and DeeperX and mining companies in collaboration with universities to develop AI’s enormous potential for critical minerals discovery.
Article by Dietmar Müller , Professor of Geophysics, University of Sydney ; Jo Condon , Honorary researcher, The University of Melbourne ; Julian Diaz , Exploration Geologist, University of Sydney , and Rohitash Chandra , Senior Lecturer, UNSW
This article is republished from The Conversation under a Creative Commons license. Read the original article .
The rush to commercialize AI is creating major security risks
At this year’s International Conference on Learning Representations (ICLR), a team of researchers from the University of Maryland presented an attack technique meant to slow down deep learning models that have been optimized for fast and sensitive operations. The attack, aptly named DeepSloth, targets “adaptive deep neural networks,” a range of deep learning architectures that cut down computations to speed up processing.
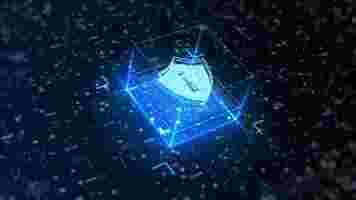
Recent years have seen growing interest in the security of machine learning and deep learning , and there are numerous papers and techniques on hacking and defending neural networks. But one thing made DeepSloth particularly interesting: The researchers at the University of Maryland were presenting a vulnerability in a technique they themselves had developed two years earlier.
In some ways, the story of DeepSloth illustrates the challenges that the machine learning community faces. On the one hand, many researchers and developers are racing to make deep learning available to different applications. On the other hand, their innovations cause new challenges of their own. And they need to actively seek out and address those challenges before they cause irreparable damage.
Shallow deep networks
One of the biggest hurdles of deep learning the computational costs of training and running deep neural networks. Many deep learning models require huge amounts of memory and processing power, and therefore they can only run on servers that have abundant resources. This makes them unusable for applications that require all computations and data to remain on edge devices or need real-time inference and can’t afford the delay caused by sending their data to a cloud server.
In the past few years, machine learning researchers have developed several techniques to make neural networks less costly. One range of optimization techniques called “multi-exit architecture” stops computations when a neural network reaches acceptable accuracy. Experiments show that for many inputs, you don’t need to go through every layer of the neural network to reach a conclusive decision. Multi-exit neural networks save computation resources and bypass the calculations of the remaining layers when they become confident about their results.
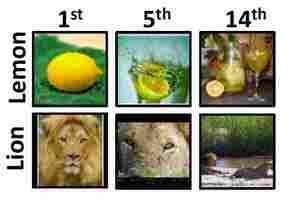
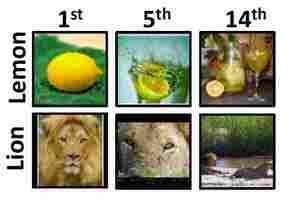
In 2019, Yigitan Kaya, a Ph.D. student in Computer Science at the University of Maryland, developed a multi-exit technique called “shallow-deep network,” which could reduce the average inference cost of deep neural networks by up to 50 percent. Shallow-deep networks address the problem of “overthinking,” where deep neural networks start to perform unneeded computations that result in wasteful energy consumption and degrade the model’s performance. The shallow-deep network was accepted at the 2019 International Conference on Machine Learning (ICML).
“Early-exit models are a relatively new concept, but there is a growing interest,” Tudor Dumitras, Kaya’s research advisor and associate professor at the University of Maryland, told TechTalks. “This is because deep learning models are getting more and more expensive computationally, and researchers look for ways to make them more efficient.”
Dumitras has a background in cybersecurity and is also a member of the Maryland Cybersecurity Center. In the past few years, he has been engaged in research on security threats to machine learning systems. But while a lot of the work in the field focuses on adversarial attacks, Dumitras and his colleagues were interested in finding all possible attack vectors that an adversary might use against machine learning systems. Their work has spanned various fields including hardware faults , cache side-channel attacks, software bugs, and other types of attacks on neural networks.
While working on the deep-shallow network with Kaya, Dumitras and his colleagues started thinking about the harmful ways the technique might be exploited.
“We then wondered if an adversary could force the system to overthink; in other words, we wanted to see if the latency and energy savings provided by early exit models like SDN are robust against attacks,” he said.
Slowdown attacks on neural networks
Dumitras started exploring slowdown attacks on shallow-deep networks with Ionut Modoranu, then a cybersecurity research intern at the University of Maryland. When the initial work showed promising results, Kaya and Sanghyun Hong, another Ph.D. student at the University of Maryland, joined the effort. Their research eventually culminated into the DeepSloth attack .
Like adversarial attacks, DeepSloth relies on carefully crafted input that manipulates the behavior of machine learning systems. However, while classic adversarial examples force the target model to make wrong predictions, DeepSloth disrupts computations. The DeepSloth attack slows down shallow-deep networks by preventing them from making early exits and forcing them to carry out the full computations of all layers.
“Slowdown attacks have the potential of negating the benefits of multi-exit architectures,” Dumitras said. “These architectures can halve the energy consumption of a deep neural network model at inference time, and we showed that for any input we can craft a perturbation that wipes out those savings completely.”
The researchers’ findings show that the DeepSloth attack can reduce the efficacy of the multi-exit neural networks by 90-100 percent. In the simplest scenario, this can cause a deep learning system to bleed memory and compute resources and become inefficient at serving users.
But in some cases, it can cause more serious harm. For example, one use of multi-exit architectures involves splitting a deep learning model between two endpoints. The first few layers of the neural network can be installed on an edge location, such as a wearable or IoT device. The deeper layers of the network are deployed on a cloud server. The edge side of the deep learning model takes care of the simple inputs that can be confidently computed in the first few layers. In cases where the edge side of the model does not reach a conclusive result, it defers further computations to the cloud.
In such a setting, the DeepSloth attack would force the deep learning model to send all inferences to the cloud. Aside from the extra energy and server resources wasted, the attack could have much more destructive impact.
“In a scenario typical for IoT deployments, where the model is partitioned between edge devices and the cloud, DeepSloth amplifies the latency by 1.5–5X, negating the benefits of model partitioning,” Dumitras said. “This could cause the edge device to miss critical deadlines, for instance in an elderly monitoring program that uses AI to quickly detect accidents and call for help if necessary.”
While the researchers made most of their tests on deep-shallow networks, they later found that the same technique would be effective on other types of early-exit models.
Attacks in real-world settings
As with most works on machine learning security, the researchers first assumed that an attacker has full knowledge of the target model and has unlimited computing resources to craft DeepSloth attacks. But the criticality of an attack also depends on whether it can be staged in practical settings, where the adversary has partial knowledge of the target and limited resources.
“In most adversarial attacks, the attacker needs to have full access to the model itself, basically, they have an exact copy of the victim model,” Kaya told TechTalks. “This, of course, is not practical in many settings where the victim model is protected from outside, for example with an API like Google Vision AI.”
To develop a realistic evaluation of the attacker, the researchers simulated an adversary who doesn’t have full knowledge of the target deep learning model. Instead, the attacker has a surrogate model on which he tests and tunes the attack. The attacker then transfers the attack to the actual target. The researchers trained surrogate models that have different neural network architectures, different training sets, and even different early-exit mechanisms.
“We find that the attacker that uses a surrogate can still cause slowdowns (between 20-50%) in the victim model,” Kaya said.
Such transfer attacks are much more realistic than full-knowledge attacks, Kaya said. And as long as the adversary has a reasonable surrogate model, he will be able to attack a black-box model, such as a machine learning system served through a web API.
“Attacking a surrogate is effective because neural networks that perform similar tasks (e.g., object classification) tend to learn similar features (e.g., shapes, edges, colors),” Kaya said.
Dumitras says DeepSloth is just the first attack that works in this threat model, and he believes more devastating slowdown attacks will be discovered. He also pointed out that, aside from multi-exit architectures, other speed optimization mechanisms are vulnerable to slowdown attacks. His research team tested DeepSloth on SkipNet, a special optimization technique for convolutional neural networks (CNN). Their findings showed that DeepSloth examples crafted for multi-exit architecture also caused slowdowns in SkipNet models.
“This suggests that the two different mechanisms might share a deeper vulnerability, yet to be characterized rigorously,” Dumitras said. “I believe that slowdown attacks may become an important threat in the future.”
Security culture in machine learning research
The researchers also believe that security must be baked into the machine learning research process.
“I don’t think any researcher today who is doing work on machine learning is ignorant of the basic security problems. Nowadays even introductory deep learning courses include recent threat models like adversarial examples,” Kaya said.
The problem, Kaya believes, has to do with adjusting incentives. “Progress is measured on standardized benchmarks and whoever develops a new technique uses these benchmarks and standard metrics to evaluate their method,” he said, adding that reviewers who decide on the fate of a paper also look at whether the method is evaluated according to their claims on suitable benchmarks.
“Of course, when a measure becomes a target, it ceases to be a good measure,” he said.
Kaya believes there should be a shift in the incentives of publications and academia. “Right now, academics have a luxury or burden to make perhaps unrealistic claims about the nature of their work,” he says. If machine learning researchers acknowledge that their solution will never see the light of day, their paper might be rejected. But their research might serve other purposes.
For example, adversarial training causes large utility drops, has poor scalability, and is difficult to get right, limitations that are unacceptable for many machine learning applications. But Kaya points out that adversarial training can have benefits that have been overlooked, such as steering models toward becoming more interpretable .
One of the implications of too much focus on benchmarks is that most machine learning researchers don’t examine the implications of their work when applied to real-world settings and realistic settings.
“Our biggest problem is that we treat machine learning security as an academic problem right now. So the problems we study and the solutions we design are also academic,” Kaya says. “We don’t know if any real-world attacker is interested in using adversarial examples or any real-world practitioner in defending against them.”
Kaya believes the machine learning community should promote and encourage research in understanding the actual adversaries of machine learning systems rather than “dreaming up our own adversaries.”
And finally, he says that authors of machine learning papers should be encouraged to do their homework and find ways to break their own solutions, as he and his colleagues did with the shallow-deep networks. And researchers should be explicit and clear about the limits and potential threats of their machine learning models and techniques.
“If we look at the papers proposing early-exit architectures, we see there’s no effort to understand security risks although they claim that these solutions are of practical value,” he says. “If an industry practitioner finds these papers and implements these solutions, they are not warned about what can go wrong. Although groups like ours try to expose potential problems, we are less visible to a practitioner who wants to use an early-exit model. Even including a paragraph about the potential risks involved in a solution goes a long way.”
This article was originally published by Ben Dickson on TechTalks , a publication that examines trends in technology, how they affect the way we live and do business, and the problems they solve. But we also discuss the evil side of technology, the darker implications of new tech, and what we need to look out for. You can read the original article here .
Instagram will automatically hide offensive comments on your posts using AI
On its 10th anniversary, Instagram is rolling out a bunch of visual features including allowing you to change the app icon. With that, the company is also introducing some measures to reduce abusive comments on the platform.
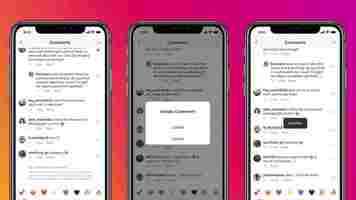
The first feature is automatically blocking offensive comments using AI. The company said that it’ll detect these by using previously reported offensive comments. You can tap on “View Hidden Comments” to see them. The social network will automatically remove all comments that violate its community guidelines. This feature is currently in a beta mode.
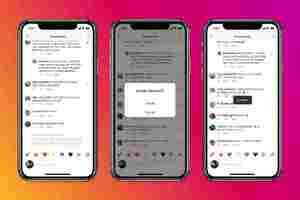
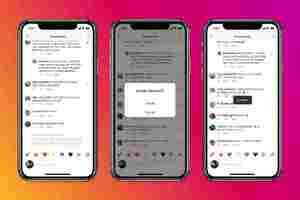
In addition to this, the company is expanding its “nudge” feature that notifies someone when they’re posting a nasty comment. Earlier, it used to prompt the offensive poster only once. Now, the app will show also warnings when someone repeatedly tries to post insulting stuff.
Instagram didn’t expand on any numbers, but it said that since rolling out the nudge feature last year, it has seen “ a meaningful decrease in negative interactions” in the comments section.
The new tools will be available globally when people are writing comments in English, Portuguese, Spanish, French, Russian, Chinese, and Arabic (Android only) to start. Instagram said it’ll expand these features to other languages in the future.