Facebook AI boss Yann LeCun goes off in Twitter rant, blames talk radio for hate content
Yann LeCun, Facebook’s world-renowned AI guru, had some problems with an article written about his company yesterday. So he did what any of us would do, he went on social media to air his grievances.
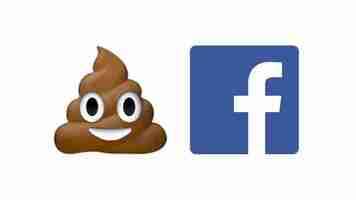
Only, he didn’t take the fight to Facebook as you’d expect. Instead, over a period of hours, he engaged in a back-and-forth with numerous people on Twitter.
Can we just stop for a moment and appreciate that, on a random Thursday in March, the father of Facebook’s AI program gets on Twitter to argue about a piece from journalist Karen Hao, an AI reporter for MIT’s Technology Review?
Hao wrote an incredible long-form feature on Facebook’s content moderation problem. The piece is called “How Facebook got addicted to spreading misinformation,” and the sub-heading is a doozy:
I’ll quote just a single paragraph from Hao’s article here that captures its essence:
There’s a lot to unpack there, but the gist is that Facebook is driven by the singular goal of “growth.” The same could be said of cancer.
LeCun, apparently, didn’t like the article. He hopped on the app that Jack built and shared his thoughts, including what appears to be personal attacks questioning Hao’s journalistic integrity:
His umbrage yesterday extended to blaming talk radio and journalism for his company’s woes:
Really Yann? Increased polarization via disinformation is uniquely American? Have you met my friend “the reason why every single war ever has been fought in the history of ever?”
I digress.
This wouldn’t be the first time he’s taken to Twitter to argue in defense of his company, but there was more going on yesterday than meets the eye. LeCun’s tirade began with a tweet announcing new research on fairness from the Facebook Artificial Intelligence Team (FAIR).
According to Hao, Facebook coordinated the release of the paper to coincide with the Tech Review article:
Based on the evidence, it appears Facebook was absolutely gobsmacked by Hao’s reporting. It seems the social network was expecting a feature on the progress its made in shoring up its algorithms, detecting bias, and combating hate speech. Instead, Hao laid bare the essential problem with Facebook: it’s a spider web.
Those are my words, not Hao’s. What they wrote was:
If I were to rephrase that for impact, I might say something like “regardless whether our company pours gasoline on the ground and offers everyone a book of matches, we’re still going to have forest fires.” But, again, those are my words.
And when I say that Facebook is a spiderweb, what I mean is: spiderwebs are good, until they become too far-reaching. For example, if you see a spiderweb in the corner of your barn, that’s great! It means you’ve got a little arachnid warrior helping you keep nastier bugs out. But if you see a spiderweb covering your entire city, like something out of “Kingdom of the Spiders,” that’s a really bad thing.
And it’s evident that LeCun knows this because his entire Twitter spiel yesterday was just one giant admission that Facebook is beyond anyone’s control. Here’s a few tidbits from his tweets on the subject:
Interesting. LeCun’s core assertion seems to be that stopping misinformation is really hard . Well, that’s true. There are a lot of things that are really hard that we haven’t figured out.
As my colleague Matthew Beedham pointed out in today’s Shift newsletter , building a production automobile that’s fueled by a nuclear reactor in its trunk is really hard.
But, as the scientists working on exactly that for the Ford company realized decades ago, nuclear technology simply isn’t advanced enough to make it safe enough to power consumer production vehicles. Nuclear’s great for aircraft carriers and submarines, but not so much for the family station wagon.
I’d argue that Facebook’s impact on humanity is almost certainly far, far more detrimental and wide-reaching than a measly little nuclear meltdown in the trunk of a Ford Mustang. After all, only 31 people died as a direct result of the Chernobyl nuclear meltdown and experts figure a max of around 4,000 were indirectly affected (health-wise, anyway).
Facebook has 2.45 billion users. And every time its platform creates or exacerbates a problem for one of those users, its answer is one version or another of “we’ll look into it.” The only place this kind of reactionary response to technology imbalance actually serves the public is in a Whac-A-Mole game.
If Facebook were a nuclear power plant trying to fix a leak that sent nuclear waste into our drinking water every time someone misused the power grid: we’d shut it down until it plugged the leaks.
But we don’t shut Facebook down because it’s not really a business. It’s a trillion-dollar PR machine for a self-governing entity. It’s a country . And we need to either sanction it or treat it as a hostile force until it does something to prevent misuses of its platform instead of only reacting when the poop hits the fan.
And, if we can’t keep the nuclear waste out of our drinking water, or build a safe car with a nuclear reactor in its trunk, maybe we ought to just shut the plants or scuttle the plans until we can. It worked out okay for Ford.
Maybe, just maybe, the reason why journalists like Hao and myself, and politicians around the globe can’t offer solutions to the problems Facebook has is because there aren’t any.
Perhaps hiring the smartest AI researchers on the planet and surrounding them with the world’s greatest PR machine isn’t enough to overcome the problem of humans poisoning each other for fun and profit on a giant unregulated social network.
There are some problems you can’t just throw money and press releases at.
My hat’s off to Karen Hao for such excellent reporting and to the staff of Technology Review for speaking truth in the face of power.
Adversarial attacks are a ticking time bomb, but no one cares
If you’ve been following news about artificial intelligence, you’ve probably heard of or seen modified images of pandas and turtles and stop signs that look ordinary to the human eye but cause AI systems to behave erratically. Known as adversarial examples or adversarial attacks , these images—and their audio and textual counterparts —have become a source of growing interest and concern for the machine learning community.
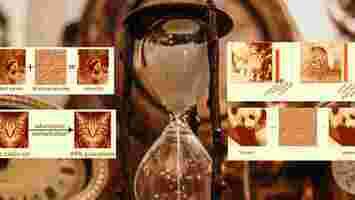
But despite the growing body of research on adversarial machine learning , the numbers show that there has been little progress in tackling adversarial attacks in real-world applications.
The fast-expanding adoption of machine learning makes it paramount that the tech community traces a roadmap to secure the AI systems against adversarial attacks. Otherwise, adversarial machine learning can be a disaster in the making.
What makes adversarial attacks different?
Every type of software has its own unique security vulnerabilities, and with new trends in software, new threats emerge. For instance, as web applications with database backends started replacing static websites, SQL injection attacks became prevalent. The widespread adoption of browser-side scripting languages gave rise to cross-site scripting attacks. Buffer overflow attacks overwrite critical variables and execute malicious code on target computers by taking advantage of the way programming languages such as C handle memory allocation. Deserialization attacks exploit flaws in the way programming languages such as Java and Python transfer information between applications and processes. And more recently, we’ve seen a surge in prototype pollution attacks , which use peculiarities in the JavaScript language to cause erratic behavior on NodeJS servers.
In this regard, adversarial attacks are no different than other cyberthreats. As machine learning becomes an important component of many applications , bad actors will look for ways to plant and trigger malicious behavior in AI models.
What makes adversarial attacks different, however, is their nature and the possible countermeasures. For most security vulnerabilities, the boundaries are very clear. Once a bug is found, security analysts can precisely document the conditions under which it occurs and find the part of the source code that is causing it. The response is also straightforward. For instance, SQL injection vulnerabilities are the result of not sanitizing user input. Buffer overflow bugs happen when you copy string arrays without setting limits on the number of bytes copied from the source to the destination.
In most cases, adversarial attacks exploit peculiarities in the learned parameters of machine learning models. An attacker probes a target model by meticulously making changes to its input until it produces the desired behavior. For instance, by making gradual changes to the pixel values of an image, an attacker can cause the convolutional neural network to change its prediction from, say, “turtle” to “rifle.” The adversarial perturbation is usually a layer of noise that is imperceptible to the human eye.
(Note: in some cases, such as data poisoning , adversarial attacks are made possible through vulnerabilities in other components of the machine learning pipeline, such as a tampered training data set.)
The statistical nature of machine learning makes it difficult to find and patch adversarial attacks. An adversarial attack that works under some conditions might fail in others, such as a change of angle or lighting conditions. Also, you can’t point to a line of code that is causing the vulnerability because it spread across the thousands and millions of parameters that constitute the model.
Defenses against adversarial attacks are also a bit fuzzy. Just as you can’t pinpoint a location in an AI model that is causing an adversarial vulnerability, you also can’t find a precise patch for the bug. Adversarial defenses usually involve statistical adjustments or general changes to the architecture of the machine learning model.
For instance, one popular method is adversarial training, where researchers probe a model to produce adversarial examples and then retrain the model on those examples and their correct labels. Adversarial training readjusts all the parameters of the model to make it robust against the types of examples it has been trained on. But with enough rigor, an attacker can find other noise patterns to create adversarial examples.
The plain truth is, we are still learning how to cope with adversarial machine learning. Security researchers are used to perusing code for vulnerabilities. Now they must learn to find security holes in machine learning that are composed of millions of numerical parameters.
Growing interest in adversarial machine learning
Recent years have seen a surge in the number of papers on adversarial attacks. To track the trend, I searched the arXiv preprint server for papers that mention “adversarial attacks” or “adversarial examples” in the abstract section. In 2014 , there were zero papers on adversarial machine learning. In 2020 , around 1,100 papers on adversarial examples and attacks were submitted to arxiv.
Adversarial attacks and defense methods have also become a key highlight of prominent AI conferences such as NeurIPS and ICLR. Even cybersecurity conferences such as DEF CON, Black Hat, and Usenix have started featuring workshops and presentations on adversarial attacks.
The research presented at these conferences shows tremendous progress in detecting adversarial vulnerabilities and developing defense methods that can make machine learning models more robust. For instance, researchers have found new ways to protect machine learning models against adversarial attacks using random switching mechanisms and insights from neuroscience .
It is worth noting, however, that AI and security conferences focus on cutting edge research. And there’s a sizeable gap between the work presented at AI conferences and the practical work done at organizations every day.
The lackluster response to adversarial attacks
Alarmingly, despite growing interest in and louder warnings on the threat of adversarial attacks, there’s very little activity around tracking adversarial vulnerabilities in real-world applications.
I referred to several sources that track bugs, vulnerabilities, and bug bounties. For instance, out of more than 145,000 records in the NIST National Vulnerability Database, there are no entries on adversarial attacks or adversarial examples. A search for “machine learning” returns five results. Most of them are cross-site scripting (XSS) and XML external entity (XXE) vulnerabilities in systems that contain machine learning components. One of them regards a vulnerability that allows an attacker to create a copy-cat version of a machine learning model and gain insights, which could be a window to adversarial attacks. But there are no direct reports on adversarial vulnerabilities. A search for “deep learning” shows a single critical flaw filed in November 2017. But again, it’s not an adversarial vulnerability but rather a flaw in another component of a deep learning system.
I also checked GitHub’s Advisory database, which tracks security and bug fixes on projects hosted on GitHub. Search for “adversarial attacks,” “adversarial examples,” “machine learning,” and “deep learning” yielded no results. A search for “TensorFlow” yields 41 records, but they’re mostly bug reports on the codebase of TensorFlow. There’s nothing about adversarial attacks or hidden vulnerabilities in the parameters of TensorFlow models.
This is noteworthy because GitHub already hosts many deep learning models and pretrained neural networks .
Finally, I checked HackerOne, the platform many companies use to run bug bounty programs. Here too, none of the reports contained any mention of adversarial attacks.
While this might not be a very precise assessment, the fact that none of these sources have anything on adversarial attacks is very telling.
The growing threat of adversarial attacks
Automated defense is another area that is worth discussing. When it comes to code-based vulnerabilities Developers have a large set of defensive tools at their disposal.
Static analysis tools can help developers find vulnerabilities in their code. Dynamic testing tools examine an application at runtime for vulnerable patterns of behavior. Compilers already use many of these techniques to track and patch vulnerabilities. Today, even your browser is equipped with tools to find and block possibly malicious code in client-side script.
At the same time, organizations have learned to combine these tools with the right policies to enforce secure coding practices. Many companies have adopted procedures and practices to rigorously test applications for known and potential vulnerabilities before making them available to the public. For instance, GitHub, Google, and Apple make use of these and other tools to vet the millions of applications and projects uploaded on their platforms.
But the tools and procedures for defending machine learning systems against adversarial attacks are still in the preliminary stages. This is partly why we’re seeing very few reports and advisories on adversarial attacks.
Meanwhile, another worrying trend is the growing use of deep learning models by developers of all levels. Ten years ago, only people who had a full understanding of machine learning and deep learning algorithms could use them in their applications. You had to know how to set up a neural network, tune the hyperparameters through intuition and experimentation, and you also needed access to the compute resources that could train the model.
But today, integrating a pre-trained neural network into an application is very easy.
For instance, PyTorch, which is one of the leading Python deep learning platforms, has a tool that enables machine learning engineers to publish pretrained neural networks on GitHub and make them accessible to developers. If you want to integrate an image classifier deep learning model into your application, you only need a rudimentary knowledge of deep learning and PyTorch.
Since GitHub has no procedure to detect and block adversarial vulnerabilities, a malicious actor could easily use these kinds of tools to publish deep learning models that have hidden backdoors and exploit them after thousands of developers integrate them in their applications.
How to address the threat of adversarial attacks
Understandably, given the statistical nature of adversarial attacks, it’s difficult to address them with the same methods used against code-based vulnerabilities. But fortunately, there have been some positive developments that can guide future steps.
The Adversarial ML Threat Matrix , published last month by researchers at Microsoft, IBM, Nvidia, MITRE, and other security and AI companies, provides security researchers with a framework to find weak spots and potential adversarial vulnerabilities in software ecosystems that include machine learning components. The Adversarial ML Threat Matrix follows the ATT&CK framework, a known and trusted format among security researchers.
Another useful project is IBM’s Adversarial Robustness Toolbox, an open-source Python library that provides tools to evaluate machine learning models for adversarial vulnerabilities and help developers harden their AI systems.
These and other adversarial defense tools that will be developed in the future need to be backed by the right policies to make sure machine learning models are safe. Software platforms such as GitHub and Google Play must establish procedures and integrate some of these tools into the vetting process of applications that include machine learning models. Bug bounties for adversarial vulnerabilities can also be a good measure to make sure the machine learning systems used by millions of users are robust.
New regulations for the security of machine learning systems might also be necessary. Just as the software that handles sensitive operations and information is expected to conform to a set of standards, machine learning algorithms used in critical applications such as biometric authentication and medical imaging must be audited for robustness against adversarial attacks.
As the adoption of machine learning continues to expand, the threat of adversarial attacks is becoming more imminent. Adversarial vulnerabilities are a ticking timebomb. Only a systematic response can defuse it.
This article was originally published by Ben Dickson on TechTalks , a publication that examines trends in technology, how they affect the way we live and do business, and the problems they solve. But we also discuss the evil side of technology, the darker implications of new tech and what we need to look out for. You can read the original article here .
How the laws of physics could prevent AI from gaining sentience
A renowned theoretical computer science expert recently released an astonishing physics pre-print paper that tosses fuel on the fiery debate over… whether humans could use wormholes to traverse the universe or not.
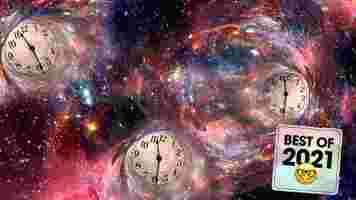
Don’t worry, I’ll explain what this has to do with self-aware robots in due course.
Fun with physics
First, however, let’s lay the foundation for our speculation with a quick glance at this all-new wormhole theory.
The pre-print paper comes courtesy of French researcher Pascal Koiran. According to them, if you apply a different theoretical math metric to our understanding of gravity at the edge of a black hole, you get a different theoretical output. Whodathunkit?
Per an article by astrophysicist Paul Sutter on LiveScience:
The implications
Until now, the Schwartzchild interpretation of black holes has made it seem like wormholes would be intraversible by any form of matter – the old “ nothing can escape a black hole, not even light” chestnut .
But the new theory says otherwise. And that seems like it should be awesome. In a few thousands years our species might be capable of journeying to the edge of time, space, and reality using magical wormhole portal guns à la Rick Sanchez .
But let’s take a closer look at the research, shall we?
Per Koiran’s pre-print paper :
The two different methods for simulating the potential path of a particle traversing a wormhole may require completely different interpretations of how time works in our universe.
If nothing can escape a blackhole, we can assume the entrance to every wormhole is permanently stuck on infinite pause in both time and space.
However, if we assume that something can escape a black hole, we may need to rethink our entire understanding of space-time.
In a universe where time itself can escape a black hole through discrete physical processes, some of our assumptions about observer theory (which states that particles, when observed, behave as waves) could be flawed.
Get to the AI stuff
Modern artificial neural networks , like the kinds that power deepfakes technology, GPT-3, and facial recognition systems, are a rudimentary attempt to imitate the machinations of the organic neural network running inside our human brains.
The ultimate goal is achieving human-level AI, also known as artificial general intelligence (AGI). However, the world’s foremost leading experts can’t quite agree on exactly how we’re supposed to achieve this.
It’s impossible to tell if we’re actually making progress towards AGI. It could happen tomorrow, in 100 years, or never.
One educated guess we can make, however, is that it’s unlikely we’ll get there with a binary neural network running classical algorithms.
We live in a quantum universe . Whether you believe in wormholes or not is inconsequential to the fact that any attempt at recreating the human brain’s organic neural network through binary representation is unlikely to result in a functional facsimile.
It’s a quantum world after all
But even an advanced quantum neural network could fail to produce AGI if the laws of physics prevent it. What if there’s no way to make a machine experience the passage of time?
Our current understanding of time is essential to how we interpret the math of physics. For instance, the unit of measurement called a “meter” that we apply to distance is currently defined by how far light travels in a vacuum in 1/299,792,458 th of a second.
So how far is a meter at the edge of a black hole? In a universe where discrete units of time-space can’t escape a black hole, the space between two points in the event horizon of singularity is operationally infinite.
The physics surrounding this version of our universe would imply that time can be disrupted. And, like all permutable things in our universe, it should be subject to observer theory.
In essence, by defining a quantum AI, we might be producing the necessary observations to manifest a temporal wave in our robot’s processing power. And that would, theoretically, mean the machine could experience a singular moment of self-awareness. Hence the term “AI singularity.”
In this version of the universe, we’re rooting for a paradigm where nothing can escape a black hole.
Here’s why: (theoretically, at least) time has to either be a construct of reality – we observe stuff, those observations are sequenced, those sequences are continuously measured in retrospect, we agree time has passed – or it has to be a discrete “thing” that exists in the universe as tangibly as protons and electrons do.
If it can escape a black hole, that indicates it’s observer-independent and, thus, likely discrete.
In a universe where space-time is as real as atoms, the trick to sentience might involve discovering a method by which to tap in to space-time’s ground truth in the same way humans apparently do.
You know how some apps won’t work if your computer’s time and date aren’t set properly? That, but for the entire universe.
Another way to put this would be: you can call it the missing piece, the quantum question, or a soul… but a universe where time itself exists independent of our observations is one where, for whatever reason, our particular biology is inexplicably special.
Far out right?
Then again, maybe Koiran is wrong. Maybe the laws of physics make it theoretically impossible to traverse a wormhole. Maybe they don’t even exist!
In which case, no, you can’t have the last 10 minutes of your life back.
But you can read the research in full here .
Further reading:
Physicists suggest there’s an ‘anti-universe’ behind ours
Theoretical physicists think humans are screwing up the universe’s plan
There’s a tiny star spraying antimatter all over the Milky Way — should we be worried?