Hey, space nerd! Here’s what Mars sounds like
We’ve long known what Mars looks like, but the Perseverance rover is finally teaching us how it sounds.
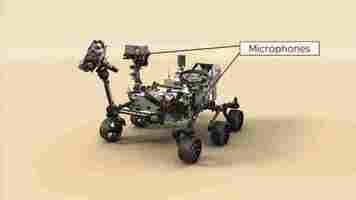
The buggy shared the first audio of the Martian surface in February and has gone on to record five hours of the planet’s sounds .
NASA this week released an array of audible delights captured by the six-wheeled spacecraft.
The sounds of gusting winds, rattling wheels, and whirring motors provide a new perspective on Mars. They also play key a role in Perseverance’s science mission.
Perseverance is equipped with two microphones, both of which are off-the-shelf devices. One sits on the buggy’s chassis and listens to the wheels and internal systems of the rover. The other mic is attached to the spacecraft’s mast.
As Perseverance’s laser instrument shoots rocks and studies the resulting plasma, the mast mic records the zaps.
The mic’s position allows it to monitor minute shifts in air that offer insights into the Martian atmosphere.
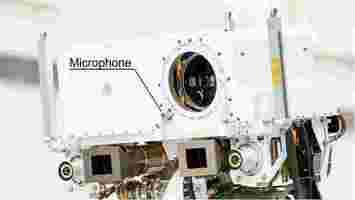
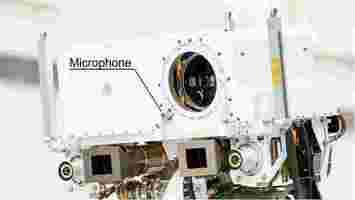
The mast mic is also providing clues about how sound propagates on the planet. Some scientists were surprised when it picked up the Ingenuity helicopter’s buzzing rotors , because high-pitched sounds are hard to hear through Mars’ thin atmosphere.
“Sound on Mars carries much farther than we thought,’ said Nina Lanza, a SuperCam scientist, in a statement. “It shows you just how important it is to do field science.”
As well as supporting scientists, the recordings can give us all new ways to experience the red planet.
Greetings Humanoids! Did you know we have a newsletter all about AI? You can subscribe to it right here .
Who writes better essays: College students or GPT-3?
The GPT-3 text generator has proven adept at producing code , blogs , and, err, bigotry . But is the AI a good student?
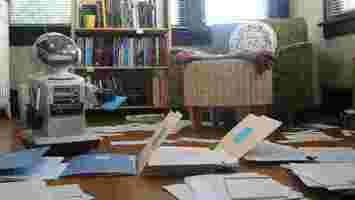
Education resource site EduRef has tried to find out — by testing the system’s essay-writing skills .
The company hired a panel of professors to create writing prompts for essays on US history, research methods, creative writing, and law.
They fed the prompts to GPT-3, and also gave them to a group of recent college graduates and undergrad students.
The anonymized papers were then marked by the panel, to test whether AI can get better grades than human pupils.
Some of the results could unnerve professors — and excite unscrupulous students. But others showed GPT-3 still has a lot to learn.
GPT-3’s highest grades were B-minuses for a history essay on American exceptionalism and a policy memo for a law class.
Its human rivals earned similar marks for their history papers: a B and a C+. But only one of three students got a higher grade than the AI for the law assignment.
GPT-3 also received a solid C for its research methods paper on COVID-19 vaccine efficacy, while the students got a B and a D.
However, the AI’s creative writing abilities couldn’t match its technical skills. Its story received the model’s solitary fail, while the student writers’ grades ranged from A to D+.
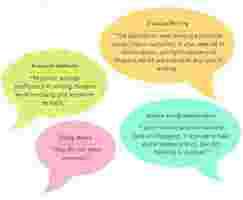
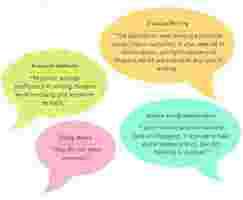
Overall, GPT-3 showed an impressive grasp of grammar, syntax, and word frequency. But it failed to craft a strong narrative for the creative writing assignment.
Project manager Sam Larson told TNW that this could be due to how GPT-3 recalls information:
Still, what GPT-3 lacked in craft it made up for in speed. The model spent between three and 20 minutes generating content for each assignment, while the humans took three days on average.
Assessing the assessment
EduRef stressed that the experiment was only an exploratory study. GPT-3’s outputs were lightly edited for length and repetition, although its content, factual information, and grammar were left untouched.
In addition, the AI produced two papers for the history, research, and law assignments. Larson then picked which ones to use:
Larson said the creative writing task required additional human interference:
Larson — who is himself an academic — was nonetheless impressed by GPT’s performance. He hopes that this type of AI-generated content gives instructors and policy-makers pause for thought about how they quantify what makes a successful student.
But students may be more interested in AI’s ability to lend them a devious helping-hand.
4 ideas about AI that even ‘experts’ get wrong
The history of artificial intelligence has been marked by repeated cycles of extreme optimism and promise followed by disillusionment and disappointment . Today’s AI systems can perform complicated tasks in a wide range of areas, such as mathematics, games, and photorealistic image generation. But some of the early goals of AI like housekeeper robots and self-driving cars continue to recede as we approach them.
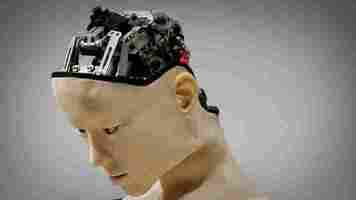
Part of the continued cycle of missing these goals is due to incorrect assumptions about AI and natural intelligence, according to Melanie Mitchell, Davis Professor of Complexity at the Santa Fe Institute and author of Artificial Intelligence: A Guide For Thinking Humans .
In a new paper titled “ Why AI is Harder Than We Think ,” Mitchell lays out four common fallacies about AI that cause misunderstandings not only among the public and the media, but also among experts. These fallacies give a false sense of confidence about how close we are to achieving artificial general intelligence, AI systems that can match the cognitive and general problem-solving skills of humans.
Narrow AI and general AI are not on the same scale
The kind of AI that we have today can be very good at solving narrowly defined problems . They can outmatch humans at Go and chess, find cancerous patterns in x-ray images with remarkable accuracy, and convert audio data to text. But designing systems that can solve single problems does not necessarily get us closer to solving more complicated problems. Mitchell describes the first fallacy as “Narrow intelligence is on a continuum with general intelligence.”
“If people see a machine do something amazing, albeit in a narrow area, they often assume the field is that much further along toward general AI,” Mitchell writes in her paper.
For instance, today’s natural language processing systems have come a long way toward solving many different problems, such as translation, text generation , and question-answering on specific problems. At the same time, we have deep learning systems that can convert voice data to text in real-time. Behind each of these achievements are thousands of hours of research and development (and millions of dollars spent on computing and data). But the AI community still hasn’t solved the problem of creating agents that can engage in open-ended conversations without losing coherence over long stretches. Such a system requires more than just solving smaller problems; it requires common sense, one of the key unsolved challenges of AI.
The easy things are hard to automate
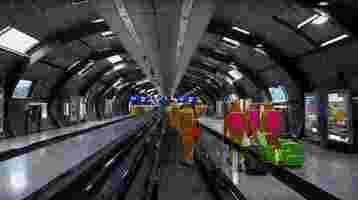
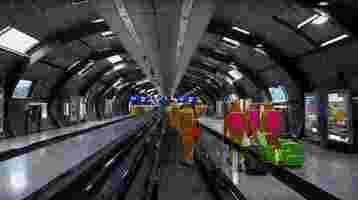
When it comes to humans, we would expect an intelligent person to do hard things that take years of study and practice. Examples might include tasks such as solving calculus and physics problems, playing chess at grandmaster level, or memorizing a lot of poems.
But decades of AI research have proven that the hard tasks, those that require conscious attention, are easier to automate. It is the easy tasks, the things that we take for granted, that are hard to automate. Mitchell describes the second fallacy as “Easy things are easy and hard things are hard.”
“The things that we humans do without much thought—looking out in the world and making sense of what we see, carrying on a conversation, walking down a crowded sidewalk without bumping into anyone—turn out to be the hardest challenges for machines,” Mitchell writes. “Conversely, it’s often easier to get machines to do things that are very hard for humans; for example, solving complex mathematical problems, mastering games like chess and Go , and translating sentences between hundreds of languages have all turned out to be relatively easier for machines.”
Consider vision, for example. Over billions of years, organisms have developed complex apparatuses for processing light signals. Animals use their eyes to take stock of the objects surrounding them, navigate their surroundings, find food, detect threats, and accomplish many other tasks that are vital to their survival. We humans have inherited all those capabilities from our ancestors and use them without conscious thought. But the underlying mechanism is indeed more complicated than large mathematical formulas that frustrate us through high school and college.
Case in point: We still don’t have computer vision systems that are nearly as versatile as human vision. We have managed to create artificial neural networks that roughly mimic parts of the animal and human vision system, such as detecting objects and segmenting images. But they are brittle, sensitive to many different kinds of perturbations, and they can’t mimic the full scope of tasks that biological vision can accomplish . That’s why, for instance, the computer vision systems used in self-driving cars need to be complemented with advanced technology such as lidars and mapping data.
Another area that has proven to be very difficult is sensorimotor skills that humans master without explicit training. Think of the how you handle objects, walk, run, and jump. These are tasks that you can do without conscious thought. In fact, while walking, you can do other things, such as listen to a podcast or talk on the phone. But these kinds of skills remain a large and expensive challenge for current AI systems.
“AI is harder than we think, because we are largely unconscious of the complexity of our own thought processes,” Mitchell writes.
Anthropomorphizing AI doesn’t help
The field of AI is replete with vocabulary that puts software on the same level as human intelligence. We use terms such as “learn,” “understand,” “read,” and “think” to describe how AI algorithms work. While such anthropomorphic terms often serve as shorthand to help convey complex software mechanisms, they can mislead us to think that current AI systems work like the human mind.
Mitchell calls this fallacy “the lure of wishful mnemonics” and writes, “Such shorthand can be misleading to the public trying to understand these results (and to the media reporting on them), and can also unconsciously shape the way even AI experts think about their systems and how closely these systems resemble human intelligence.”
The wishful mnemonics fallacy has also led the AI community to name algorithm-evaluation benchmarks in ways that are misleading. Consider, for example, the General Language Understanding Evaluation (GLUE) benchmark , developed by some of the most esteemed organizations and academic institutions in AI. GLUE provides a set of tasks that help evaluate how a language model can generalize its capabilities beyond the task it has been trained for. But contrary to what the media portray, if an AI agent gets a higher GLUE score than a human, it doesn’t mean that it is better at language understanding than humans.
“While machines can outperform humans on these particular benchmarks, AI systems are still far from matching the more general human abilities we associate with the benchmarks’ names,” Mitchell writes.
A stark example of wishful mnemonics is a 2017 project at Facebook Artificial Intelligence Research, in which scientists trained two AI agents to negotiate on tasks based on human conversations. In their blog post , the researchers noted that “updating the parameters of both agents led to divergence from human language as the agents developed their own language for negotiating [emphasis mine].”
This led to a stream of clickbait articles that warned about AI systems that were becoming smarter than humans and were communicating in secret dialects. Four years later, the most advanced language models still struggle with understanding basic concepts that most humans learn at a very young age without being instructed.
AI without a body