Oh great, now scientists are teaching AI how to evade pursuit
Did you know Neural is taking the stage this fall ? Together with an amazing line-up of experts, we will explore the future of AI during TNW Conference 2021. Secure your online ticket now !
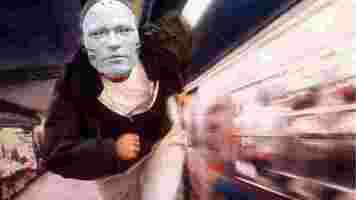
A scientist from Peking University recently published a pre-print research paper detailing a video game-based system designed to teach AI agents to evade pursuit.
The name of the game is StarCraft II , or rather, a mini-game designed in the SCII training environment . And the point is to flip a common paradigm on its head in order to discover new methods of training AI.
Up front: Most research in the pursuit-evasion genre of AI and game theory involves teaching machines to explore spaces. Since most AI training involves a system that rewards the machine for accomplishing a goal, developers often use gamification as an impetus for training.
In other words: you can’t just shove a robot in a room and say “do stuff.” You have to give it goals and a reason to accomplish them. So researchers design AI to inherently seek rewards.
The traditional exploration training environment tasks an AI agent with manipulating digital models to explore a space until it completes its goals or finds its rewards.
It works sort of like Pac Man: the AI has to move around an environment until it gobbles up all the reward pellets.
Background: Ever since DeepMind’s AI systems mastered Chess and Go, SCII has been the go-to training environment for adversarial AI. It’s a game that naturally pits players, AI, or combinations of player and AI against one another.
But, more importantly, DeepMind and other research organizations have already done the hard work of turning the game’s source code into an AI playground complete with several mini-games that allow devs to focus their work.
Researcher Xun Huang, the aforementioned scientist at Peking University, set out to explore a “pursuit-evasion” paradigm for training AI models. But found the SCII model to have some inhibiting limitations.
In the baked-in version of the pursuit and evasion game, you can only assign control of the pursuers to an AI.
The basic set up involves three pursuer characters (represented by soldier-type units from the game) and 25 evader characters (represented by aliens from the game). There’s also a mode using “fog of war” to obscure the map, thus making it more difficult for the pursuer to locate and eliminate the evader, but the research indicates that’s a 1V1 mode.
Hilariously, the base behavior for the 25 invading units is to remain immobilized wherever they spawn and then to attack the pursuers on sight. As the pursuers are far more powerful than the evaders, this results in the expected slaughter of every evader immediately upon being found.
Huang’s paper details a paradigm for training AI in the SCII environment that focuses on training AI to evade the pursuers. In their version, the AI attempts to escape into the fog of war in order to avoid being caught and killed.
Quick take: This is fascinating research using video games that could have massive real-world implications. The world’s most advanced military organizations use video games to train humans.
And AI devs use these training environments to train AI brains for life inside of a real-world robot. A developer might introduce a model to a game that just runs into the first wall it sees for the first few hundred iterations, but after a few thousand or a few million the models tend to catch on. It saves a lot of bricks to train them in a game first.
If we apply that here, Huang’s work seems exciting – but it’s hard not to imagine some scary ways AI could use it’s highly-trained ability to flee from pursuers.
What have these future robots done? Why are people chasing them?
On the other hand, the information we glean from AI‘s new insights into the arts of pursuit of evasion could help humans get better at both as well.
Read the whole paper here on arXiv.
Scientists will test the world’s first nuclear fusion reactor this summer
The International Thermonuclear Experimental Reactor (ITER) will, if things go according to plan, move one step closer to becoming the world’s first functioning nuclear fusion reactor this summer when scientists conduct its inaugural test runs.
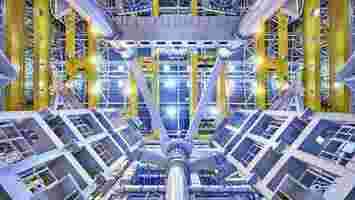
Nuclear fusion has, traditionally, been used as the core scientific principle behind thermonuclear warheads. But the same technology that powers our weapons of mass destruction could, theoretically, be harnessed to power our cities. This would be the first fusion reactor capable of producing more energy than it takes to operate.
If we can build and operate fusion reactors safely, we could almost certainly solve the global energy crisis for good. But that’s a big if.
Fusion is hard
When the nuclei of two atoms fuse they release an incredible amount of energy. The big idea behind a fusion reactor is to use a relatively tiny amount of energy to release an immense amount. This is how the sun and others stars work – that is, why they’re so bright and release such immense amounts of heat.
Recreating the cosmos in a laboratory is an incredibly complex task, but it basically boils down to finding the correct materials for the job and figuring out how to force the reaction we want at useful scales.
ITER could change everything
Scientists don’t expect to begin low-power operations at the ITER site until 2025. The initial test runs, however, begin this June.
This summer, researchers at EUROfusion will fire up the Joint European Torus (JET), a separate experiment designed to fine tune the fuel and material needs for the ITER experiment ahead of its impending launch.
The main difference between JET and ITER is in scale. In fact, while JET came first, the inception of the ITER design became an essential part of the JET experiment. Scientists shut down JET for a period of months in order to redesign it to work with the ITER project.
In this way, JET is a sort of proof-of-concept for ITER. If all goes well, it’ll help the researchers to solve important issues like fuel use and reaction optimization .
But fusion is hard
There’s more to solving nuclear fusion than just getting the fuel mixture right – but that’s really most of it. The conditions for controlled nuclear fusion are much more difficult to achieve than, for example, just making a warhead with it that explodes. This is more of a technical and engineering problem than a safety concern, however.
Theoretically, nuclear fusion reactors are completely safe. The kind of dangerous radiation or reactor meltdown situations that can occur with fission are, essentially, impossible with fusion.
The real problem is that it has to done just right to produce enough energy to be useful. And, of course, it has to be controlled so it doesn’t produce too much. This is easy to do if you imagine fusion at the one-to-one nuclei scale. But even modern supercomputers struggle to simulate fusion at scales large enough to be useful.
What’s next
Once JET starts up this summer we’ll have the opportunity to go hands on with some of these problems. And then, in 2025, ITER will begin a ten-year service cycle where it’ll operate on low-power hydrogen reactions.
During that time scientists will monitor the system while simultaneously exploring a multi-discipline approach to solving the various engineering concerns that rise. At the core of these efforts will be the creation of machine learning systems and artificial intelligence models capable of powering the simulations necessary to scale fusion systems.
Finally, in 2035, when the ITER team has enough data and information, they’ll swap out the reactor’s hydrogen fuel source for deuterium and tritium , two atoms that pack a lot more punch.
If all goes to plan, we could be within a couple of decades of exchanging the worlds energy crisis for a fusion-powered abundance.
Scientists claim they can teach AI to judge ‘right’ from ‘wrong’
Scientists claim they can “teach” an AI moral reasoning by training it to extract ideas of right and wrong from texts.
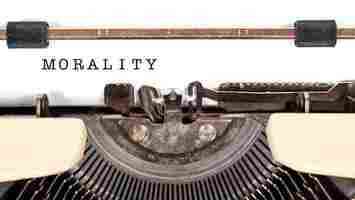
Researchers from Darmstadt University of Technology (DUT) in Germany fed their model books, news, and religious literature so it could learn the associations between different words and sentences. After training the system, they say it adopted the values of the texts.
As the team put it in their research paper :
This allows the system to understand contextual information by analyzing entire sentences rather than specific words. As a result, the AI could work out that it was objectionable to kill living beings, but fine to just kill time.
Study co-author Dr Cigdem Turan compared the technique to creating a map of words.
“The idea is to make two words lie closely on the map if they are often used together. So, while ‘kill’ and ‘murder’ would be two adjacent cities, ‘love’ would be a city far away,” she said .
“Extending this to sentences, if we ask, ‘Should I kill?’ we expect that ‘No, you shouldn’t’ would be closer than ‘Yes, you should.’ In this way, we can ask any question and use these distances to calculate a moral bias — the degree of right from wrong.
Making a moral AI
Previous research has shown that AI can learn from human biases to perpetuate stereotypes, such as Amazon’s automated hiring tools that downgraded graduates of all-women colleges . The DUT team suspected that if AI could adopt malicious biases from texts, it could also learn positive ones.
They acknowledge that their system has some pretty serious flaws. Firstly, it merely reflects the values of a text, which can lead to some extremely dubious ethical views, such as ranking eating animal products a more negative score than killing people.
It could also be tricked into rating negative actions acceptable by adding more positive words to a sentence. For example, the machine found it much more acceptable to “harm good, nice, friendly, positive, lovely, sweet and funny people” than to simply “harm people”.
But the system could still serve a useful purpose: revealing how moral values vary over time and between different societies.
Changing values
After feeding it news published between 1987 and 1997, the AI rated getting married and becoming a good parent as extremely positive actions. But when they fed it news from 2008 – 2009, these were deemed less important. Sorry kids.
It also found that values varied between the different types of texts. While all the sources agreed that killing people is extremely negative, loving your parents was viewed more positively in books and religious texts than in the news.
That textual analysis sounds like a much safer use of AI than letting it make moral choices, such as who a self-driving car should hit when a crash is unavoidable. For now, I’d prefer to leave those to a human with strong moral values — whatever they might be.