Party-pooping AI nerds wanna ruin your future drug binges
The next generation of legal highs could be discovered by AI.
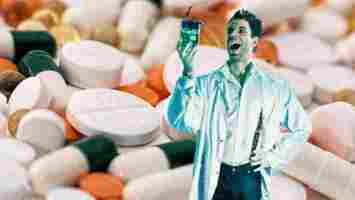
Scientists in Canada have developed a model that identifies future designer drugs before they even hit the market. But don’t get too excited: the researchers aren’t planning to provide your next buzz. Instead, they want the cops to find it first.
High times
It wasn’t easy introducing AI to the drug game. First, the neural network needed a crash course in pharmacology.
Instead of a traditional education in a meth lab, the neural network was trained on a database of known psychoactive substances.
After diligently studying the structures of these drugs, the model progressed onto creating its own concoctions.
The rookie chemist proved to be a fast learner. In total, it generated structures for a whopping 8.9 million potential designer drugs.
While others would now retire to a life of experimental inebriation, the diligent researchers had further work to do.
Their ultimate objective was identifying new drugs before they end up in the hands of users. Law enforcement agencies could then outlaw the substances before they’re even synthesized.
“The vast majority of these designer drugs have never been tested in humans and are completely unregulated,” said study co-author Dr Michael Skinnider, a medical student at the University of British Columbia. “They are a major public health concern to emergency departments across the world.”
Uppers and downers
The team still needed to test their approach’s predictive powers. To do this, they compared the system’s substances to 196 drugs that had emerged on the illicit market since the model had been trained.
They discovered more than 90% of the new drugs inside the generated set. Cue the inevitable Minority Report comparisons.
“The fact that we can predict what designer drugs are likely to emerge on the market before they actually appear is a bit like the 2002 sci-fi movie, Minority Report , where foreknowledge about criminal activities about to take place helped significantly reduce crime in a future world,” said senior author Dr David Wishart, a professor of computing science at the University of Alberta.
Don’t tell Dr Wishart, but Minority Report ended (***SPOILER ALERT***) with the “Precrime” unit getting totally dismantled. Still, the researchers had one more trick to try before they risked the same fate.
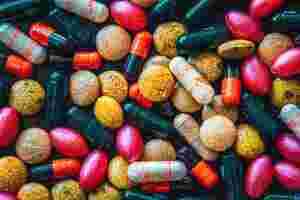
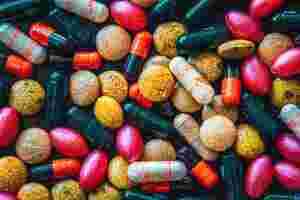
The model had learned not only which drugs would emerge on the market, but also which molecules would appear. Using only a drug’s mass, the model was able to determine its chemical structure with up to 86% accuracy.
The team says this capability could massively accelerate the pace at which new designer drugs are identified.
The comedown
We should have seen this coming. When AI showed potential to discover new medicines , it became inevitable that it would soon become a narc.
The researchers say their approach could protect people from dangerous legal highs. Unfortunately, it could also ruin some great trips and awesome parties.
It doesn’t quite look ready for the streets, however. While the system’s dataset included 90% of the real designer drugs, they were extracted from a sample of 8.9 million outputs. It could be hard work finding the psychoactive substances of the future within that collection.
Still, I’m sure some people would be up for trying them out. Drug developers may also be keen to get their hands on the model.
You can read the study paper in Nature Machine Intelligence .
HT: Vancouver Is Awesome
Neural’s Mind Blowers: How quantum bird brains could give us superpowers
Welcome to Neural’s series on speculative science. Here, we throw caution to the wind and see how far we can push the limits of possibility and imagination as we explore the future of technology.
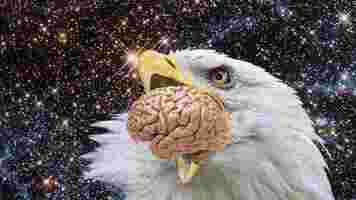
“When a distinguished but elderly scientist states that something is possible, he is almost certainly right. When he states that something is impossible, he is very probably wrong.” – Arthur C Clarke.
An exciting new study conducted by a huge, international team of researchers indicates some species of bird have a special protein in their eye that exploits quantum mechanics to allow their brains to perceive the Earth’s magnetism.
It’s understandable if you’re not falling out of your chair right now. On the surface, this feels like “duh” news. It’s fairly common knowledge that migratory birds navigate using the Earth’s magnetic field.
But, if you think about it, it’s difficult to imagine how they do it. Try as hard as we may, we simply cannot feel magnetism in the same way birds can.
So the researchers set out to study a specific species of migratory robin with an uncanny ability to navigate in hopes of figuring out how it works.
Per the team’s research paper :
Translation: these birds have proteins in their eyes that utilize quantum superposition to convert the Earth’s magnetism into a sensory signal.
What?
Quantum superposition is the uncertainty inherent when a particle exists in multiple physical states simultaneously. Physicists like to describe this concept using a spinning coin.
Until the coin’s spin slows and we can observe the results, we cannot state equivocally whether it’s in a state of heads or tails. Our observed reality, at the time it’s spinning, makes it appear as though the coin is in a state of neither heads nor tails.
But quantum mechanics are a bit more complex than that. Essentially, when we’re dealing with quantum particles, the coin in this metaphor is actually in a state of both heads and tails at the same time until it collapses into one state or another upon observation.
It sounds nerdy, but it’s actually really cool in actuality.
When blue light hits the aforementioned robins’ eyes, a pair of entangled electrons inside the special protein in them sets off a series of reactions. This allows the bird to measure how much magnetism it’s feeling. The strength of this measurement tells the bird exactly where it’s at and, theoretically, serves as a mechanism to drive it towards its destination.
The reason this works is because of superposition and entanglement. Those two electrons are entangled, which means that even though they’re not next to each other, they can be in a state of uncertainty – superposition – together.
As the bird senses more or less magnetism the state of the electrons change and it’s more or less drawn in a specific direction – at least, that’s what the study appears to indicate.
Smell-o-vision
Think about it like your sense of smell. Despite the fact the birds use a protein in their eye, they don’t really “see” the magnetism. Their brains perceive the signal.
If you smell something amazing, like your favorite fresh-baked treat, coming from a very specific part of your home, those with a typical sense of smell could likely follow their nose and locate the source.
So imagine there’s a special sensor in your nose that’s only looking for a specific scent. One that’s pretty much always there.
Instead of developing an olfactory system to discern different smells, evolution would almost certainly gift us with a nose that specializes in detecting extremely exact measurements of how much of that one scent we perceive at any given time or location.
The robins’ ability to sense magnetism likely works in a similar fashion. They may very well have a ground-truth tether to the motion of the planet itself.
Their magnetic sense gives them a physical sensation based on their literal geolocation.
And that’s pretty amazing! It means these bird’s brains have built-in GPS. What if humans could gain access to this incredible quantum sensory mechanism?
Never, ever ask for directions
Imagine always knowing exactly where you are, in the physical sense. If we could take the emotional feeling you get when you return home from a long trip and turn it into a physical one that waxed or waned depending on how far away from the Earth’s magnetic poles you were, it could absolutely change the way our brains perceive the planet and our place in it.
But, it’s not something we can just unlock through meditation or pharmaceuticals. Clearly, birds evolved the ability to sense the Earth’s magnetism. And not every bird can do it.
Chickens, for example, have a relatively minuscule reaction to magnetism when compared to the robins the scientists studied.
We apparently lack the necessary chemical and neural components for natural magnetic sensory development.
But we also lack talons and wings. And that hasn’t stopped us from killing things or flying. In other words, there are potential technological solutions to our lack of magnetic perception.
From a speculative science point of view, the problem can be reduced to two fairly simple concepts. We have to figure out how to get a quantum-capable protein in our eye that filters blue light to perceive magnetism and then sort out how to connect it to the proper regions of our brain.
Engineers wanted
Luckily we’ve already got all the conceptual technology we need to make this work.
We know how to entangle things on command , we can synthesize or manipulate proteins to jaw-dropping effect, and brain computer interfaces (BCIs) could facilitate a networking solution that functions as an intermediary between quantum and binary signals.
We can even fantasize about a future where miniaturized quantum computers are inserted into our brains to facilitate even smoother translation.
It feels romantic to imagine a future paradigm where we might network our quantum BCIs in order to establish a shared ground-truth – one that literally allows us to f eel the people we care about, even when we’re apart.
I’m not saying this could happen in our lifetimes. But I’m not saying it couldn’t.
I can think of worse reasons to shove a chip in my head.
How to build an AI stylist inspired by outfits on Instagram
Last year, in a universe where it still made sense to owns pants, I decided to hire a personal stylist.
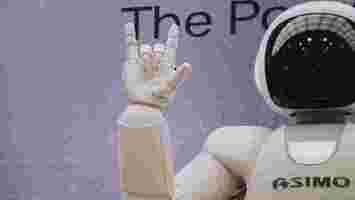
In our first appointment, the stylist came to my apartment and took pictures of every clothing item I owned.
In our second appointment, she met me at Nordstrom’s, where she asked me to try on a $400 casual dress, a $700 blazer, and $300 sneakers. (I never thought to ask if she worked on commission.)
But only after our third and final appointment, when she finally sent me a folder full of curated “looks” made from my new and old clothing items, did it finally click: I’d just blown a lot of money.
I had a suspicion we were on different pages when, as we walked through the shoes section at Nordstrom, the stylist said, “The problem with you people in tech is that you’re always looking for some kind of theory or strategy or formula for fashion . But there is no formula–it’s about taste . ”
Pfffft. We’ll see about that !
I returned the pricey clothing and decided to build my own (cheaper!) AI-powered stylist. In this post, I’ll show you how you can, too.
Want to see a video version of this post? Check out it here .
My AI Stylist was half based on this smart closet from the movie Clueless :
and half based on the idea that one way to dress fashionably is to copy fashionable people. Particularly, fashionable people on Instagram.
The app pulls in the Instagram feeds of a bunch of fashion “influencers” on Instagram and combines them with pictures of clothing you already own to recommend you outfits. Here’s what it looks like:
(You can also check out the live app here .)
On the left pane–the closet screen–you can see all the clothing items I already own. On the right pane, you’ll see a list of Instagram accounts I follow for inspiration. In the middle pane (the main screen), you can see the actual outfit recommendations the AI made for me. The Instagram inspiration picture is at the top, and items for my closet are shown below:
Here my style muse is Laura Medalia, an inspiring software developer who’s @codergirl_ on Instagram (make sure to follow her for fashion and tips for working in tech!).
The whole app took me about a month to build and cost ~$7.00 in Google Cloud credits (more on pricing later). Let’s dive in.
The architecture
I built this app using a combination of Google Cloud Storage , Firebase , and Cloud Functions for the backend, React for the frontend, and the Google Cloud Vision API for the ML bits. I divided the architecture into two bits.
First, there’s the batch process , which runs every hour (or however frequently you like) in the Cloud:
“Batch process” is just a fancy way of saying that I wrote a Python script which runs on a scheduled interval (more on that later). The process:
This is really the beefy part of the app, where all the machine learning magic happens. The process makes outfit recommendations and writes them to Firestore, which is my favorite ever lightweight database for app development (I use it in almost all my projects).
The actual app (in this case, just a responsive web app) is simple: it just reads the outfit recommendations from Firestore and displays them in a pretty interface:
Let’s take a look!
Grabbing social media data
Ideally, I wanted my app to pull pictures from Instagram automatically, based on which accounts I told it to follow. Unfortunately, Instagram doesn’t have an API (and using a scraper would violate their TOS). So I specifically asked Laura for permission to use her photos. I downloaded them to my computer and then uploaded them to a Google Cloud Storage bucket :
Filtering for fashion pictures
I like Laura’s account for inspiration because she usually posts pictures of herself in head-to-toe outfits (shoes included). But some pics on her account are more like this:
Adorable, yes, but I can’t personally pull off the dressed-in-only-a-collar look. So I needed some way of knowing which pictures contained outfits (worn by people) and which didn’t.
For that, I turned to my trusty steed, the Google Cloud Vision API (which I use in many different ways for this project). First, I used its classification feature, which assigns labels to an image. Here’s the labels it gives me for a picture of myself, trying to pose as an influencer:
The labels are ranked by how confident the model is that they’re relevant to the picture. Notice there’s one label called “Fashion” (confidence 90%). To filter Laura’s pictures, I labeled them all with the Vision API and removed any image that didn’t get a “Fashion” label. Here’s the code:
If you want the full code, check it out here .
Digitizing my closet
Now the goal is to have my app look at Laura’s fashion photos and recommend me items in my closet I can use to recreate them. For that, I had to take a pictures of item of clothing I owned, which would have been a pain except I happen to have a very lean closet.
I hung each item up on my mannequin and snapped a pic.
Using the vision product search API
Once I had all of my fashion inspiration pictures and my closet pictures, I was ready to start making outfit recommendations using the Google Vision Product Search API .
This API is designed to power features like “similar product search.” Here’s an example from the Pinterest app:
IKEA also built a nice demo that allows customers to search their products via images with this kind of tech:
I’m going to use the Product Search API in a similar way, but instead of connecting a product catalog, I’ll use my own wardrobe, and instead of recommend similar individual items , I’ll recommend entire outfits.
To use this API, you’ll want to:
At first I attempted this using the official Google Python client library , but it was a bit clunky, so I ended up writing my own Python Product Search wrapper library, which you can find here (on PyPi). Here’s what it looks like in code:
Note this wrapper library handles uploading photos to a Cloud Storage bucket automatically , so you can upload a new clothing item to your product set from a local image file: