Researchers developed ‘explainable’ AI to help diagnose and treat at-risk children
A pair of researchers from the Oak Ridge Laboratory have developed an “explainable” AI system designed to aid medical professionals in the diagnosis and treatment of children and adults who’ve experienced childhood adversity. While this is a decidedly narrow use-case, the nuts and bolts behind this AI have particularly interesting implications for the machine learning field as a whole.
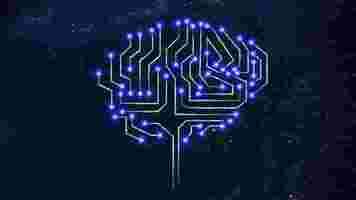
Plus, it represents the first real data-driven solution to the outstanding problem of empowering general medical practitioners with expert-level domain diagnostic skills – an impressive feat in itself.
Let’s start with some background. Adverse childhood experiences (ACEs) are a well-studied form of medically relevant environmental factors whose effect on people, especially those in minority communities, throughout the entirety of their lives has been thoroughly researched.
While the symptoms and outcomes are often difficult to diagnose and predict, the most common interventions are usually easy to employ. Basically: in most cases we know what to do with people suffering from or living in adverse environmental conditions during childhood, but we often don’t have the resources to take these individuals completely through the diagnosis to treatment pipeline.
Enter Nariman Ammar and Arash Shaban-Nejad, two medical researchers from the University of Tennesee’s Oak Ridge National Laboratory. They today published a pre-print paper outlining the development and testing of a novel AI framework designed to aid in the diagnosis and treatment of individuals meeting the ACEs criteria.
Unlike a broken bone, ACEs aren’t diagnosed through physical examinations. They require a caretaker or medical professional with training and expertise in the field of childhood adversity to diagnose. While the general gist of diagnosing these cases involves asking patients questions, it’s not so simple as just going down a checklist.
Medical professionals may not suspect ACEs until the “right” questions are asked, and even then the follow-up questions are often more insightful. Depending on the particular nuances of an individual case, there could be tens of thousands of potential parameters (combinations of questions and answers) affecting the recommendations for intervention a healthcare provider may need to make.
And, perhaps worse, once interventions are made – meaning, appointments are set with medical, psychiatric, or local/government agencies that can aid the patient – there’s no guarantees the next person in the long line of healthcare and government workers a patient will encounter is going to be as competent when it comes to understanding ACEs as the last one.
The Oak Ridge team’s work is, in itself, an intervention. It’s designed to work much like a tech support chat bot. You input patient information and it recommends and schedules interventions based on the various databases its trained on.
This may sound like a regular chatbot, but this AI makes a lot of inferences. It processes plain language requests such as “my home has no heating” into inferences about childhood adversity (housing issues) and then searches through what’s essentially the computer-readable version of a medical textbook on ACEs and decides on the best course of action to recommend to a medical professional.
The Q&A isn’t a pre-scripted checklist, but instead a dynamic conversation system based on “Fulfillments” and webhooks that, according to the paper, “enable the agent to invoke external service endpoints and send dynamic responses based on user expressions as opposed to hard-coding those responses.”
Using its own inferences, it decides which questions to ask based on context from previously answered ones. The goal here is to save time and make it as frictionless as possible to extrapolate the most useful information possible in the least amount of questions.
Coupled with end-level scheduling abilities, this could end up being a one-stop-shop for helping people who, otherwise, may continue living in an environment that could cause permanent, lifelong damage to their health and well-being.
The best part about this AI system is that it’s fully explainable. It converts those fulfillment and webhooks into actionable items by attaching them to the relevant snippets of data it used to extrapolate its end-results. This, according to the research, allows for an open-box fully traceable system that – barring any eventual UI and connectivity issues – should be usable by anyone.
If this methodology can be applied to other domains – like, for example, making it less painful to deal with just about every other chatbot on the planet – it could be a game changer for the already booming service bot industry .
As always keep in mind that arXiv papers are preprints that haven’t been peer-reviewed and they’re subject to change or retraction. You can read more about the Oak Ridge team’s new AI framework here .
China’s ‘Wu Dao’ AI is 10X bigger than GPT-3, and it can sing
China’s going all in on deep learning. The Beijing Academy of Artificial Intelligence (BAAI) recently released details concerning its “Wu Dao” AI system – and there’s a lot to unpack here.
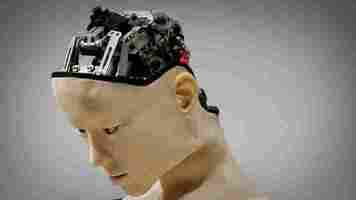
Up front: Wu Dao is a multi-modal AI system. That means it can do a bunch of different things. It can generate text, audio, and images, and, according to Engadget , it can even “power virtual idols.”
The reason for all the hullabaloo surrounding Wu Dao involves its size. This AI model is huge . It was trained using a whopping 1.75 trillion parameters. For comparison, OpenAI’s biggest model, GPT-3, was trained with just 175 billion.
Background: According to Zhang Hongjiang, the chairman of BAAI, the academy’s intent is to create the biggest, most powerful AI model possible.
Per the aforementioned Engadget report, Zhang said:
Quick take: This AI system sounds like a breakthrough UI for deep learning tricks, but it’s doubtful this kind of brute-force method will eventually lead to general artificial intelligence.
It’s cool to know there’s a powerful AI out there that can make music videos, write poetry, and create captions for images on its own. And, with so many parameters, Wu Dao surely produces some incredibly convincing outputs.
But creating a general AI – that is, an AI capable of performing any relative task a human can – isn’t necessarily a matter of increasing the power and parameters of a deep learning system.
Details as to exactly how Wu Dao was trained, what was in its various datasets, and what practical applications it can be used for remain scarce. It’s impossible to do a direct comparison to GPT-3 at this point.
But, even if we assume Wu Dao is 10-times better across the board, there’s still no reason to believe that’ll move the needle any closer to truly-intelligent machines.
A steadily-increasing number of AI and computer science experts are under the belief that deep learning is a dead-end street for general artificial intelligence.
We may already be seeing diminishing returns on power if the most exciting thing about a system trained on supercomputer clusters with 1.75T parameters is that it can generate digital pop stars.
AI can’t tell if you’re lying – anyone who says otherwise is selling something
Another day, another problematic AI study. Today’s snake oil special comes via Tel Aviv University where a team of researchers have unveiled a so-called “lie-detection system.”
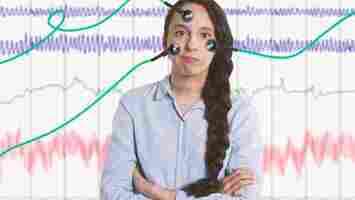
Let’s be really clear right up front: AI can’t do anything a person, given an equivalent amount of time to work on the problem, couldn’t do themselves. And no human can tell if any given human is lying. Full stop.
The simple fact of the matter is that some of us can tell when some people are lying some of the time. Nobody can tell when anybody is lying all of the time.
The university makes the following claim via press release :
That’s a really weird statement. The idea that “73%” accuracy at detecting lies is indicative of a particular paradigm’s success is arguable at best.
What exactly is accuracy?
Base luck gives any system capable of choice a 50/50 chance. And, traditionally , that’s about how well humans perform at guessing lies. Interestingly, they perform much better at guessing truths. Some studies claim humans achieve about the same “accuracy” at determining truth statements as the Tel Aviv team’s “lie-detection system” does at determining truthfulness.
The Tel Aviv University team’s paper even mentions that polygraphs aren’t admissible in courts because they’re unreliable. But they fail to point out that polygraph devices (which have been around since 1921 ) beat their own system in so-called “accuracy” — polygraphs average about an 80% – 90% accuracy-rate in studies.
But let’s take a deeper look at the Tel Aviv team’s study anyway. The team started with 48 participants, 35 of which were identified as “female.” Six participants were cut because of technical issues, two got dropped for “never lying,” and one participated in “only 40 out of 80 trials when monetary incentives were not presented.”
So, the data for this study was generated from two sources: a proprietary AI system and 39-40 human participants. Of those participants, an overwhelming majority were identified as “female,” and there’s no mention of racial, cultural, or religious diversity.
Furthermore, the median age of participants was 23 and there’s no way to determine if the team considered financial backgrounds, mental health, or any other concerns.
All we can tell is that a small group of people averaging 23-years in age, mostly “female,” paired off to participate in this study.
There was also compensation involved. Not only were they paid for their time, which is standard in the world of academia research, but they were also paid for successfully lying to humans.
That’s a red flag. Not because it’s unethical to pay for study data (it isn’t). But because it’s adding unnecessary parameters in order to intentionally or ignorantly muddy up the study.
The researchers explain this by claiming it was part of the experiment to determine whether incentivization changed people’s ability to lie.
But, with such a tiny study sample, it seems ludicrous to cram the experiment full of needless parameters. Especially ones that are so half-baked they couldn’t possibly be codified without solid background data.
How much impact does a financial incentive have on the efficacy of a truth-telling study? That sounds like something that needs its own large-scale study to determine.
Let’s just move on to the methodology
The researchers paired off participants into liars and receivers. The liars put on headphones and listened for either the word “tree” or “line” and then were directed to either tell the truth or lie about which they’d heard. Their partner’s job was to guess if they were being lied to.
The twist here is that the researchers created their own electrode arrays and attached them to the liars’ faces and then developed an AI to interpret the outputs. The researchers operated under an initial assumption that twitches in our facial muscles are a window to the ground-truth.
This assumption is purely theoretical and, frankly, ridiculous. Stroke victims exist. Bell’s Palsy exists. Neurodiverse communication exists. Scars and loss of muscle strength exist. At least 1 billion people in the world currently live with some form of physical disability and nearly as many live with a diagnosed mental disorder.
Yet, the researchers expect us to believe they’ve invented a one-size-fits-all algorithm for understanding humans. They’re claiming they’ve stumbled across a human trait that inextricably links the mental act of deceit with a singular universal physical expression. And they accomplished this by measuring muscle twitches in the faces of just 40 humans?
Per the aforementioned press release:
So the big idea here is to generate data with one experimental paradigm (physical electrodes) in order to develop a methodology for a completely different experimental paradigm (computer vision)? And we’re supposed to believe that this particular mashup of disparate inputs will result in a system that can determine a human’s truthfulness to such a degree that its outputs are admissible in court?
That’s a bold leap to make! The team may as well be claiming it’s solved AGI with black box deep learning. Computer vision already exists. Either the data from the electrodes is necessary or it isn’t.
What’s worse, they apparently intend to develop this into a snake oil solution for governments and big businesses.
The press release continues with a quote:
Police interrogations? Airports? What?
Exactly what percentage of those 40 study participants were Black, Latino, disabled, autistic, or queer? How can anyone, in good faith and conscience, make such grandiose scientific claims about AI based on such a tiny sprinkling of data?
If this “AI solution” were to actually become a product, people could potentially be falsely arrested, detained at airports, denied loans, and passed over for jobs because they don’t look, sound, and act exactly like the people who participated in that study.
This AI system was only able to determine whether someone was lying with a 73% level of accuracy in an experiment where the lies were only one word long , meant nothing to the person saying them, and had no real effect on the person hearing them.
There’s no real-world scenario analogous to this experiment. And that “73% accuracy” is as meaningless as a Tarot card spread or a Magic 8-Ball’s output.
Simply put: A 73% accuracy rate over less than 200 iterations of a study involving a maximum of 20 data groups (the participants were paired off) is a conclusion that indicates your experiment is a failure.
The world needs more research like this, don’t get me wrong. It’s important to test the boundaries of technology. But the claims made by the researchers are entirely outlandish and clearly aimed at an eventual product launch.
Sadly, there’s about a 100% chance that this gets developed and ends up in use by US police officers.
Just like predictive-policing , Gaydar , hiring AI , and all the other snake oil AI solutions out there, this is absolutely harmful.
But, by all means, don’t take my word for it: read the entire paper and the researchers‘ own conclusions here .