This amazing new physics theory made me believe time travel is possible
There’s a lot of discussion about “time” in the world of quantum physics. At the micro level, where waves and particles can behave the same, time tends to be much more malleable than it is in our observable realm of classical physics.
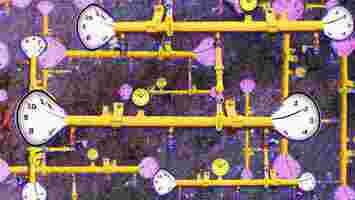
Think about the clock on the wall. You can push the hands backwards, but that doesn’t cause time itself to rewind. Time marches on.
But things are much simpler in the quantum realm. If we can mathematically express particulate activity in one direction, then we can mathematically express it in a diametric one.
In other words: time travel actually makes sense through a quantum lens. Whatever goes forward must be able to go backward.
Related: Google’s ‘time crystals’ could be the greatest scientific achievement of our lifetimes
But it all falls apart when we get back to classical physics. I don’t care how much math you do, you can’t unbreak a bottle, untell a secret, or unshoot a gun.
As Gid’on Lev points out in a recent article on Haaretz, this disparity between quantum and classical physics is one of the field’s biggest challenges.
Per Lev’s article:
Hawking was wrong, then he was right
Lev’s article goes on to explain how Stephen Hawking eventually conceded ( he lost a bet ) that the information entering a black hole wasn’t gone. He, of course, couldn’t explain exactly where it went. But most physicists were pretty sure it had to go somewhere – nothing else in the universe just vanishes.
Fast forward to 2019 and two separate research teams (working independently of each other) published pre-print papers seemingly confirming Hawking’s hunch about the persistence of information.
Not only were the papers published within 24 hours of each other, but the lead authors on each ended up sharing the 2021 New Horizons Breakthrough Prize for Fundamental Physics .
What both teams discovered was that a slight change in perspective made all the math line up.
When information enters a black hole it appears to be lost because, for all intents and purposes, it’s no longer available to the universe.
And that’s what stumped Hawking. Imagine a single photon of light getting caught in a black hole and swallowed up. Hawking and his colleagues knew the photon (and the information that was swallowed up with it) couldn’t be deleted .
But, according to Hawking, black holes leak thermal radiation. And that means they eventually lose their energy and mass and… fade away.
Hawking and company couldn’t figure out how to reconcile the fact that once a black hole is gone, anything that’s ever been inside it appears to be gone too.
That’s because they were looking in the wrong places. Hawking and others were trying to find signs of the missing information leaking out simiarly along a black hole’s event horizon .
Unfortunately, using the event horizon as a starting point never panned out – the numbers didn’t quite add up.
The 2021 New Horizons Prize winners figured out a different way to measure the “area” of a black hole. And, by applying the new lens to measurements over various stages of a black hole’s life, they were finally able to make the numbers add up.
Here’s how this relates to time travel
If these two teams did in fact demonstrate that even a black hole can’t render information irreversible, then there might be nothing physically stopping us from time travel.
And I’m not talking about that hard-to-explain, gravity at the edge of a black hole, your friends would get older while you stayed young kind of time travel.
I’m talking about real-life Marty Mcfly time travel where you could set the dials in the DeLorean for 13 March 1986 so you could go back and invest in Microsoft on the day its stock went public.
Now, much like Stephen Hawking, I don’t have any math or engineering solutions to the problem at hand. I’ve just got this physics theory.
If information can and does escape from black holes, then it’s only logical to assume that other processes which we only see in quantum mechanics could also be explained through classical physics.
We know that time travel is possible in quantum mechanics. Google demonstrated this by building time crystals , and numerous quantum computing paradigms rely on a form of prediction that surfaces answers using what’s basically molecular time-travel .
But we all know that, when it comes to quantum stuff, we’re talking about particles demonstrating counter-intuitive behavior. That’s not the same thing as pushing a button and making a car from the 1980s appear back in the old Wild West .
However, that doesn’t mean quantum time travel isn’t just as mind-blowing. Translating time crystals into something analogous in classical physics would mean creating donuts that reappear on your plate after you eat them or beer that reappears in your glass no matter how many times you chug it.
If we concede that time crystals exist and information can escape a black hole, then we have to admit that donuts – or anything, even people – could one day travel through time too.
Then again, nobody showed up for Hawking’s party . So, either it isn’t possible or time travelers are jerks.
Report: Brexit could help China and the US in the global AI race
The EU is falling further behind the US and China in the AI race, and will lose more ground after Brexit , according to a new report.
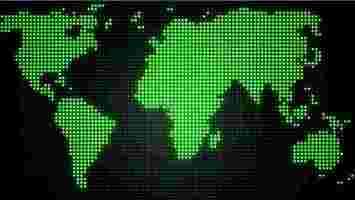
The Information Technology and Innovation Foundation assessed the trio’s AI capabilities by analyzing data across six categories: talent, research, commercial development, hardware, adoption, and data.
The think tank found that while the US still holds a substantial overall lead, China is reducing the gap in several important areas — while the EU’s position is slipping:
Of the 100 total available points, the US scooped 44.6, followed by China with 32, and the EU with 23.3.
The EU scored impressively on research quality. But it’s dropped further behind the US in number of funding deals, acquisitions of AI companies, and the amount of AI firms that have raised at least $1 million in funding.
The researchers expect the UK’s departure from the EU to further diminish the bloc’s AI capabilities. They note that British AI firms received nearly 40% of the EU’s venture and equity deals in 2019, and that five of the bloc’s 14 firms currently developing AI chips are based in the UK.
The US, meanwhile, performed strongly in areas such as startup funding and research investment. However, China has improved the quality of its own AI research.
The country has also surpassed the EU as the world leader in AI publications, and is now home to nearly twice as many of the world’s top 500 supercomputers as the US.AI
Study author Daniel Castro said the Chinese government is reaping the benefits of its strategic focus on:
What AI researchers can learn from the NFL Combine
How useful is your AI? It’s not a simple question to answer. If you’re trying to decide between Google‘s Translate service or Microsoft’s Translator, how do you know which one is better?
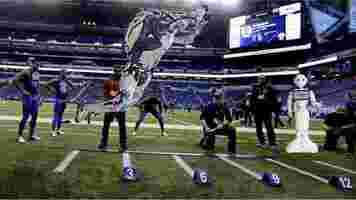
If you’re an AI developer, there’s a good chance you think the answer is: benchmarks. But that’s not the whole story.
The big idea
Benchmarks are necessary, important, and useful within the context of their own domain. If you’re trying to train an AI model to distinguish between cats and dogs in images, for example, it’s pretty useful to know how well it performs.
But since we can’t literally take our model out and use it to scan every single image of a cat and dog that’s ever existed or ever will exist, we have to sort of guess how good it will be at its job.
To do that, we use a benchmark. Basically, we grab a bunch of pictures of cats and dogs and we label them correctly. Then we hide the labels from the AI and ask it to tell us what’s in each image.
If it scores 9 out of 10, it’s 90% accurate. If we think 90% accurate is good enough, we can call our model successful. If not, we keep training and tweaking.
The big problem
How much money would you pay for an AI capable of telling a cat from a dog? A billion dollars? Half a nickel? Nothing? Probably nothing. It wouldn’t be very useful outside of the benchmark leaderboards.
However, an AI capable of labeling all the objects in any given image would be very useful.
But there’s no “universal benchmark” for labeling objects. We can only guess how good such an AI would be at its job. Just like we don’t have access to every image of a cat and dog in existence, we also cannot label everything that could possibly exist in image form.
And that means any benchmark measuring how good an AI is at labeling images is an arbitrary one.
Is an AI that’s 43% accurate at labeling images from a billion categories better or worse than one that’s 71% accurate at labeling images from 28 million categories? Does it matter what the categories are?
BD Tech Talks’ Ben Dickson put it best in a recent article :
We’re developing AI systems that are very good at passing tests, but they often fail to perform well in the real world.
The big solution
It turns out that guessing performance at scale isn’t a problem isolated to the world of AI. In 1982, National Football Scouting Inc. held the first “ NFL Combine ” to address the problems of busts – players who don’t perform as well as they were predicted to.
In the pre-internet era, the only way to evaluate players was in person and the travel expenses involved in scouting hundreds or thousands of players throughout the year were becoming too great. The Combine was a place where NFL scouts could gather to judge player performance at the same time.
Not only did this save time and money, but it also established a universal benchmark. When a team wanted to trade or release a player, other teams could refer to their “benchmark” performance at the combine.
Of course, there are no guarantees in sports. But, essentially, the Combine puts players through a series of drills that are specifically relevant to the sport of football.
However, the Combine is just a small part of the scouting process. In the modern era, teams hold private player workouts so they can determine whether a prospect appears to be a good fit in an organization’s specific system.
Another way of putting all that would be that NFL team developers use a model’s benchmarks as a general performance predictor, but they also conduct rigorous external checks to determine the model’s usefulness in a specific domain.
A player may knock your socks off at the Combine, but if they fail to impress in individual workouts there’s a pretty good chance they won’t make the team.
Ideally, benchmarking in the AI world would simply represent the first round of rigor.
As a team of researchers from UC Berkeley, the University of Washington, and Google Research recently wrote :