To achieve AGI, we need new perspectives on intelligence
This article is part of “ the philosophy of artificial intelligence ,” a series of posts that explore the ethical, moral, and social implications of AI today and in the future.
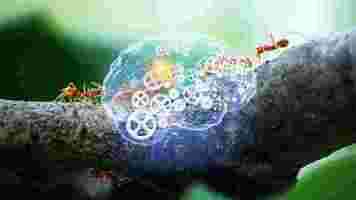
For decades, scientists have tried to create computational imitations of the brain. And for decades, the holy grail of artificial general intelligence , computers that can think and act like humans, has continued to elude scientists and researchers.
Why do we continue to replicate some aspects of intelligence but fail to generate systems that can generalize their skills like humans and animals? One computer scientist who has been working on AI for three decades believes that to get past the hurdles of narrow AI , we must look at intelligence from a different and more fundamental perspective.
In a paper that was presented at the Brain-Inspired Cognitive Architectures for Artificial Intelligence (BICA*AI), Sathyanaraya Raghavachary, Associate Professor of Computer Science at the University of Southern California, discusses “considered response,” a theory that can generalize to all forms of intelligent life that have evolved and thrived on our planet.
Titled, “ Intelligence—consider this and respond! ” the paper sheds light on the possible causes of the troubles that have haunted the AI community for decades and draws important conclusions, including the consideration of embodiment as a prerequisite for AGI.
Structures and phenomena
“Structures, from the microscopic to human level to cosmic level, organic and inorganic, exhibit (‘respond with’) phenomena on account of their spatial and temporal arrangements, under conditions external to the structures,” Raghavachary writes in his paper.
This is a general rule that applies to all sorts of phenomena we see in the world, from ice molecules becoming liquid in response to heat, to sand dunes forming in response to wind, to the solar system’s arrangement.
Raghavachary calls this “sphenomics,” a term he coined to differentiate from phenomenology, phenomenality, and phenomenalism.
“Everything in the universe, at every scale from subatomic to galactic, can be viewed as physical structures giving rise to appropriate phenomena, in other words, S->P,” Raghavachary told TechTalks.
Biological structures can be viewed in the same way, Raghavachary believes. In his paper, he notes that the natural world comprises a variety of organisms that respond to their environment. These responses can be seen in simple things such as the survival mechanisms of bacteria, as well as more complex phenomena such as the collective behavior exhibited by bees, ants, and fish as well as the intelligence of humans.
“Viewed this way, life processes, of which I consider biological intelligence—and where applicable, even consciousness—occur solely as a result of underlying physical structures,” Raghavachary said. “Life interacting with environment (which includes other life, groups…) also occurs as a result of structures (e.g., brains, snake fangs, sticky pollen…) exhibiting phenomena. The phenomena are the structures’ responses.”
Intelligence as considered response
In inanimate objects, the structures and phenomena are not explicitly evolved or designed to support processes we would call “life” (e.g., a cave producing howling noises as the wind blows by). Conversely, life processes are based on structures that consider and produce response phenomena.
However different these life forms might be, their intelligence shares a common underlying principle, Raghavachary says, one that is “simple, elegant, and extremely widely applicable, and is likely tied to evolution.”
In this respect, Raghavachary proposes in his paper that “intelligence is a biological phenomenon tied to evolutionary adaptation, meant to aid an agent survive and reproduce in its environment by interacting with it appropriately—it is one of considered response .”
The considered response theory is different from traditional definitions of intelligence and AI , which focus on high-level computational processing such as reasoning, planning, goal-seeking, and problem-solving in general. Raghavachary says that the problem with the usual AI branches— symbolic , connectionist, goal-driven—is not that they are computational but that they are digital.
“Digital computation of intelligence has—pardon the pun—no analog in the natural world,” Raghavachary said. “Digital computations are always going to be an indirect, inadequate substitute for mimicking biological intelligence – because they are not part of the S->P chains that underlie natural intelligence.”
There’s no doubt that the digital computation of intelligence has yielded impressive results, including the variety of deep neural network architectures that are powering applications from computer vision to natural language processing. But despite the similarity of their results to what we perceive in humans, what they are doing is different from what the brain does, Raghavachary says.
The “considered response” theory zooms back and casts a wider net that all forms of intelligence, including those that don’t fit the problem-solving paradigm.
“I view intelligence as considered response in that sense, emanating from physical structures in our bodies and brains. CR naturally fits within the S->P paradigm,” Raghavachary said.
Developing a theory of intelligence around the S->P principle can help overcome many of the hurdles that have frustrated the AI community for decades, Raghavachary believes. One of these hurdles is simulating the real world, a hot area of research in robotics and self-driving cars .
“Structure->phenomena are computation-free, and can interact with each other with arbitrary complexity,” Raghavachary says. “Simulating such complexity in a VR simulation is simply untenable. Simulation of S->P in a machine will always remain exactly that, a simulation.”
Embodied artificial intelligence
A lot of work in the AI field is what is known as “ brain in a vat ” solutions. In such approaches, the AI software component is separated from the hardware that interacts with the world. For example, deep learning models can be trained on millions of images to detect and classify objects. While those images have been collected from the real world, the deep learning model has not directly experienced them.
While such approaches can help solve specific problems, they will not move us toward artificial general intelligence, Raghavachary believes.
In his paper, he notes that there is not a single example of “brain in a vat” in nature’s diverse array of intelligent lifeforms. And thus, the considered response theory of intelligence suggests that artificial general intelligence requires agents that can have a direct embodied experience of the world.
“Brains are always housed in bodies, in exchange for which they help nurture and protect the body in numerous ways (depending on the complexity of the organism),” he writes.
Bodies provide brains with several advantages, including situatedness, sense of self, agency, free will, and more advanced concepts such as theory of mind (the ability to predict other the experience of another agent based on your own) and model-free learning (the ability to experience first and reason later).
“A human AGI without a body is bound to be, for all practical purposes, a disembodied ‘zombie’ of sorts, lacking genuine understanding of the world (with its myriad forms, natural phenomena, beauty, etc.) including its human inhabitants, their motivations, habits, customs, behavior, etc. the agent would need to fake all these,” Raghavachary writes.
Accordingly, an embodied AGI system would need a body that matches its brain, and both need to be designed for the specific kind of environment it will be working in.
“We, made of matter and structures, directly interact with structures, whose phenomena we ‘experience’. Experience cannot be digitally computed—it needs to be actively acquired via a body,” Raghavachary said. “To me, there is simply no substitute for direct experience.”
In a nutshell, the considered response theory suggests that suitable pairings of synthetic brains and bodies that directly engage with the world should be considered life-like, and appropriately intelligent, and—depending on the functions enabled in the hardware—possibly conscious.
This means that you can create any kind of robot and make it intelligent by equipping it with a brain that matches its body and sensory experience.
“Such agents do not need to be anthropomorphic—they could have unusual designs, structures and functions that would produce intelligent behavior alien to our own (e.g., an octopus-like design, with brain functions distributed throughout the body),” Raghavachary said. “That said, the most relatable human-level AI would likely be best housed in a human-like agent.”
This article was originally published by Ben Dickson on TechTalks , a publication that examines trends in technology, how they affect the way we live and do business, and the problems they solve. But we also discuss the evil side of technology, the darker implications of new tech, and what we need to look out for. You can read the original article here .
A scientist created emotion recognition AI for animals
A researcher at Wageningen University & Research recently published a pre-print article detailing a system by which facial recognition AI could be used to identify and measure the emotional state of farm animals. If you’re imagining a machine that tells you if your pigs are joyous or your cows are grumpy… you’re spot on.
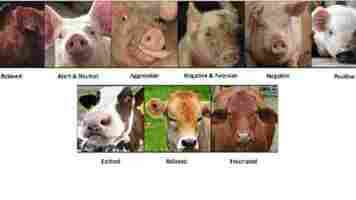
Up front: There’s little evidence to believe that so-called ’emotion recognition’ systems actually work. In the sense that humans and other creatures can often accurately recognize (as in: guess) other people’s emotions, an AI can be trained on a human-labeled data set to recognize emotion with similar accuracy to humans.
However, there’s no ground-truth when it comes to human emotion. Everyone experiences and interprets emotions differently and how we express emotion on our faces can vary wildly based on cultural and unique biological features.
In short: The same ‘ science‘ driving systems that claim to be able to tell if someone is gay through facial recognition or if a person is likely to be aggressive , is behind emotion recognition for people and farm animals.
Basically, nobody can tell if another person is gay, or aggressive just by looking at their face. You can guess. And you might be right. But no matter how many times you’re right, it’s always a guess and you’re always operating on your personal definitions.
That’s how emotion recognition works too. What you might interpret as “upset,” might just be someone’s normal expression. What you might see as “gay,” well.. I defy anyone to define internal gayism (ie: do thoughts or actions make you recognizably gay?).
It’s impossible to “train” a computer to recognize emotions because computers don’t think. They rely on data sets labeled by humans. Humans make mistakes. Worse, it’s ridiculous to imagine any two humans would look at a million faces and come to a blind consensus on the emotional state of each person viewed.
Researchers don’t train AI to recognize emotion or make inferences from faces. They train AI to imitate the perceptions of the specific humans who labeled the data they’re using.
That being said: Creating an emotion recognition engine for animals isn’t necessarily a bad thing.
Here’s a bit from the researcher’s paper :
The paper goes on to describe the system as a high-value, low-impact machine learning paradigm where farmers can gauge livestock comfort in real-time using cameras instead of invasive procedures such as hormone sampling.
We covered something similar in the agricultural world a while back . Basically, farmers operating orchards can use image recognition AI to determine if any of their trees are sickly. When you have 10s of thousands of trees, performing a visual inspection on each one of them in a timely manner is impossible for humans. But AI can stare at trees all day and night.
AI for livestock monitoring is a different beast altogether. Instead of recognizing specifically-defined signs of disease in relatively-motionless trees, the researcher’s attempting to tell what mood a bunch of animals are in.
Does it work? According to the researcher, yes. But according to the research : kinda. The paper makes claims of incredibly high accuracy, but that’s when compared against human spotters.
So here’s the thing: Creating an AI that can tell what pigs and cows are thinking almost as accurately as the world’s leading human experts is a lot like creating a food so delicious it impresses a chef. Maybe the next chef doesn’t like it, maybe nobody but that chef likes it.
The point is: this system uses AI to do a slightly poorer job than a farmer can at determining what a cow is thinking by looking at it. There’s value in that, because farmers can’t stare at cows all day and night waiting for one of them to grimace in pain.
Here’s why this is fine: Because there’s a slight potential that the animals could be treated a tiny bit better. While it’s impossible to tell exactly what an animal is feeling, the AI can certainly recognize signs of distress, discomfort, or pain well enough to make it worth while to employ this system in places where farmers could and would intervene if they thought their animals were in discomfort.
Unfortunately, the main reason why this matters is because livestock that lives in relative comfort tends to produce more.
It’s a nice fantasy to imagine a small, farm-to-table, family installing cameras all over their massive free-range livestock facility. But, more likely, systems like this will help corporate farmers find the sweet spot between packing animals in and keeping their stress levels just low enough to produce.
Final thoughts: It’s impossible to predict what the real-world use cases for this will be, and there are definitely some strong ones. But it muddies the water when researchers compare a system that monitors livestock to an emotion recognition system for humans.
Whether a cow gets a little bit of comfort before it’s slaughtered or as it spends the entirety of its life connected to dairy machinery isn’t the same class of problem as dealing with emotion recognition for humans.
Consider the fact that, for example, emotion recognition systems tend to classify Black men’s faces as angrier than white men’s . Or women, typically, rate pain higher when observing its perceived existence in people and animals. Which bias do we train the AI with?
Because, based on the current state of the technology, you can’t train an AI without bias unless the data you’re generating is never touched by human hands, and even then you’re creating a separate bias category.
You can read the whole paper here .
How Deepfakes could help implant false memories in our minds
The human brain is a complex, miraculous thing. As best we can tell, it’s the epitome of biological evolution. But it doesn’t come with any security software preinstalled. And that makes it ridiculously easy to hack.
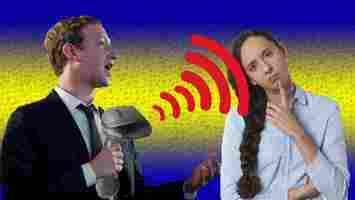
We like to imagine the human brain as a giant neural network that speaks its own language. When we talk about developing brain-computer interfaces we’re usually discussing some sort of transceiver that interprets brainwaves. But the fact of the matter is that we’ve been hacking human brains since the dawn of time.
Think about the actor who uses a sad memory to conjure tears or the detective who uses reverse psychology to draw out a suspect’s confession. These examples may seem less extraordinary than, say, the memory-eraser from Men in Black. But the end result is essentially the same. We’re able to edit the data our minds use to establish base reality. And we’re really good at it.
Background
A team of researchers from universities in Germany and the UK today published pre-print research detailing a study in which they successfully implanted and removed false memories in test subjects.
Per the team’s paper :
Basically, it’s relatively easy to implant false memories. Getting rid of them is the hard part.
The study was conducted on 52 subjects who agreed to allow the researchers to attempt to plant a false childhood memory in their minds over several sessions. After awhile, many of the subjects began to believe the false memories. The researchers then asked the subjects’ parents to claim the false stories were true.
The researchers discovered that the addition of a trusted person made it easier to both embed and remove false memories.
Per the paper :
False memory planting techniques have been around for awhile, but there hasn’t been much research on reversing them. Which means this paper comes not a moment too soon.
Enter Deepfakes
There aren’t many positive use cases for implanting false memories. But, luckily, most of us don’t really have to worry about being the target of a mind-control conspiracy that involves being slowly led to believe a false memory over several sessions with our own parents’ complicity.
Yet, that’s almost exactly what happens on Facebook every day. Everything you do on the social media network is recorded and codified in order to create a detailed picture of exactly who you are. This data is used to determine which advertisements you see, where you see them, and how frequently they appear. And when someone in your trusted network happens to make a purchase through an ad, you’re more likely to start seeing those ads.
But we all know this already right? Of course we do, you can’t go a day without seeing an article about how Facebook and Google and all the other big tech companies are manipulating us. So why do we put up with it?
Well, it’s because our brains are better at adapting to reality than we give them credit for. The moment we know there’s a system we can manipulate, the more we think the system says something about us as humans.
A team of Harvard researchers wrote about this phenomenon back in 2016:
What does this have to do with Deepfakes? It’s simple: if we’re so easily manipulated through tidbits of exposure to tiny little ads in our Facebook feed, imagine what could happen if advertisers started hijacking the personas and visages of people we trust?
You might not, for example, plan on purchasing some Grandma’s Cookies products anytime soon, but if it was your grandma telling you how delicious they are in the commercial you’re watching… you might.
Using existing technology it would be trivial for a big tech company to, for example, determine you’re a college student who hasn’t seen their parents since last December. With this knowledge, Deepfakes, and the data it already has on you, it wouldn’t take much to create targeted ads featuring your Deepfaked parents telling you to buy hot cocoa or something.
But false memories?
It’s all fun and games when the stakes just involve a social media company using AI to convince you to buy some goodies. But what happens when it’s a bad actor breaking the law? Or, worse, what happens when it’s the government not breaking the law?
Police use a variety of techniques to solicit confessions. And law enforcement are generally under no obligation to tell the truth when doing so. In fact, it’s perfectly legal in most places for cops to outright lie in order to obtain a confession.
One popular technique involves telling a suspect that their friends, families, and any co-conspirators have already told the police they know it was them who committed the crime. If you can convince someone that the people they respect and care about believe they’ve done something wrong, it’s easier for them to accept it as a fact.
How many law enforcement agencies in the world currently have an explicit policy against using manipulated media in the solicitation of a confession? Our guess would be: close to zero.
And that’s just one example. Imagine what an autocratic or iron-fisted government could do at scale with these techniques.
The best defense…
It’s good to know there are already methods we can use to extract these false memories. As the European research team discovered, our brains tend to let go of the false memories when challenged but cling to the real ones. This makes them more resilient against attack than we might think.
However it does put us perpetually on the defensive. Currently, our only defense against AI-assisted false memory implantation is to either see it coming or get help after it happens.
Unfortunately the unknown unknowns make that a terrible security plan. We simply can’t plan for all the ways a bad actor could exploit the loophole that makes it easier to edit our brains when someone we trust is helping the process along.
With Deepfakes and enough time, you could convince someone of just about anything as long as you can figure out a way to get them to watch your videos.
Our only real defense is to develop technology that sees through Deepfakes and other AI-manipulated media. With brain-computer interfaces set to hit consumer markets within the next few years and AI-generated media becoming less distinguishable from reality by the minute, we’re closing in on a point of no return for technology.
Just like the invention of the firearm made it possible for those unskilled in sword fighting to win a duel and the creation of the calculator gave those who struggle with math the ability to perform complex calculations, we may be on the cusp of an era where psychological manipulation becomes a push-button enterprise.