Trading bots: Is it game over for human financial analysts?
It’s often said that a trader’s worst enemy is himself. Behavioral biases tend to throw otherwise rational trading strategies out of whack as anxieties over loss aversion, the fear of missing out, or even overconfidence take control—ultimately putting portfolios in jeopardy. Fortunately, technology has progressed to a point where impulsive decision-making humans can be replaced by unerring and emotionally-neutral trading bots. And some believe they’re the future of finance.
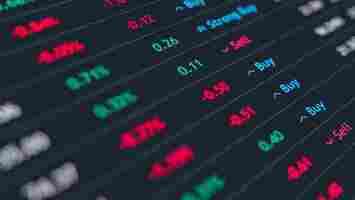
Conquering cognitive bias: A quantitative approach
When evaluating an investment, traders use several strategies to better identify entry and exit opportunities. Among them is qualitative and quantitative analysis. The latter involves statistical modeling on technical aspects such as volatility and historical performance, while the former concerns data analysis pertaining to company management, earnings, competitive advantage, and other such subjective information.
Per the 2020 PwC–Elwood Crypto Hedge Fund Report , however, it’s the quantitative approach that stands as a clear favorite among crypto fund managers. According to the report’s survey, a significant 48% of respondents claimed to use a quantitative strategy. And the rationale behind it is perfectly clear. It all boils down to eliminating cognitive biases—something which is all too prevalent in trading. This goes double for the crypto market, where volatility reigns king.
Furthermore, given the data-centric features of the cryptocurrency market (the multitude of trading venues, transaction volumes, fees, market capitalization, etc.), quantitative analysts can dig down deeper than they typically would in traditional financial assets—providing further scope for calculability and prediction.
Regardless of how refined a trader’s analytic prowess may be, cognitive bias represents an ever-present threat.
There have been multiple studies into the influence of cognitive bias in trading—and just as many tactics attempting to overcome it. Behavioral finance—a subfield of behavioral economics—argues that psychological influence is the sole reason for market irregularities, such as price crashes and parabolic upside movements.
A study administered by researchers of the MIT Sloan School of Management examined the emotional reactivity on trading performance . The report concluded that extreme emotional responses are detrimental to trader returns, particularly during volatility and times of crisis.
However, a differing, almost antithetical school of thought to behavioral finance, known as modern portfolio theory (MPT), assumes that the market is efficient and that traders are totally rational.
Neither behavioral finance nor MPT is entirely correct, but neither is wholly incorrect either. Like the yin and yang of investment, these two approaches equalize each other, providing traders with a comfortable and realistic middle ground.
However, it’s MPT’s approach to portfolio construction that truly stands out as a strategy to avoid behavioral biases, especially loss aversion bias, i favoring the avoidance of losses over potential gains. MPT argues that diversifying between multiple assets can maximize returns despite the risk-return profile of individual assets. In other words: don’t put all your eggs in one basket. This method evades loss aversion bias by offsetting risk through pairing uncorrelated assets. And it’s just one of the strategic tools in the trading bot arsenal.
Trading bots vs human researchers
Trading bots, which come in both analyst and advisor varieties, are designed to take on the traditional research advisor and analysts’ role, and often employ a mixture of the aforementioned strategies (particularly quantitative analysis and diversification) to attain their user’s goals. A typical robo advisor will build a basket of data based on the risk profile of the client, whereas robo analysts will delve into SEC filings and data released in annual company reports. But it’s their ability to combat cognitive bias amid volatile, stressful, and high-pressure market situations that place these bots a cut above the rest. And they’ve already proven to outperform their human counterparts as a result.
In December 2019, researchers from Indiana University evaluated over 76,000 research reports issued over 15 years by a range of robo-analysts. As it turns out, the robo buy recommendations outperformed those of the human analysts, granting 5% higher profit margins.
But not all robo analysts and advisors are created equal. This year, researchers measured the performance of 20 German B2C robo-advisors , assessed from May 2019 to March 2020—a time frame that serendipitously coincided with both a bull market in 2019 and the onset and fallout of the coronavirus pandemic. The disparity between the bots was tremendous, with the top robo advisor limiting downdraws to just -3.8% and outperforming the rest by around 14 basis points on average—a fairly impressive feat considering March’s market-wide double-digit collapse, which brought average year-to-date losses of 9.8% for hedge funds .
The principal difference between the top performer and the others was its strategic approach. Rather than the typical portfolio constructs, based on conventional measures of risk, the top performer measured precisely what traders are scared of: losing money and taking a long time to recover from those losses. By factoring in quantitative analysis and behavioral finance, the top performer was able to read the market, outperforming both robo advisors and human-run funds.
It comes as no surprise then that major banks are starting to turn to automated researchers. Last year, Goldman Sachs announced its own robo-advisory service . While the launch is delayed until 2021 to the coronavirus, the market for robo advisors hasn’t slowed down, with usage increasing between 50 and 30% from Q4 2019 to Q1 2020.
But given its data-rich and risk-on landscape, the crypto market is where robo analysis will truly deliver.
This article was originally published by Anton Altement on TechTalks , a publication that examines trends in technology, how they affect the way we live and do business, and the problems they solve. But we also discuss the evil side of technology, the darker implications of new tech and what we need to look out for. You can read the original article here .
We assign too much humanity to robots: They’re simply tools
In the mid-1990s, there was research going on at Stanford University that would change the way we think about computers. The Media Equation experiments were simple: participants were asked to interact with a computer that acted socially for a few minutes after which, they were asked to give feedback about the interaction .
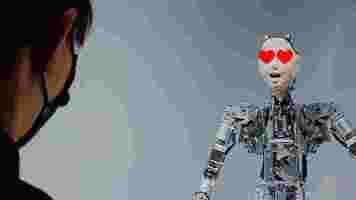
Participants would provide this feedback either on the same computer (No. 1) they had just been working on or on another computer (No. 2) across the room. The study found that participants responding on computer No. 2 were far more critical of computer No. 1 than those responding on the same machine they’d worked on.
People responding on the first computer seemed to not want to hurt the computer’s feelings to its face , but had no problem talking about it behind its back . This phenomenon became known as the computers as social actors (CASA) paradigm because it showed that people are hardwired to respond socially to technology that presents itself as even vaguely social.
The CASA phenomenon continues to be explored, particularly as our technologies have become more social. As a researcher, lecturer, and all-around lover of robotics, I observe this phenomenon in my work every time someone thanks a robot , assigns it a gender , or tries to justify its behavior using human, or anthropomorphic, rationales .
What I’ve witnessed during my research is that while few are under any delusions that robots are people, we tend to defer to them just like we would another person.
Social tendencies
While this may sound like the beginnings of a Black Mirror episode , this tendency is precisely what allows us to enjoy social interactions with robots and place them in caregiver, collaborator, or companion roles.
The positive aspects of treating a robot like a person is precisely why roboticists design them as such — we like interacting with people. As these technologies become more human-like, they become more capable of influencing us. However, if we continue to follow the current path of robot and AI deployment, these technologies could emerge as far more dystopian than utopian.
The Sophia robot, manufactured by Hanson Robotics, has been on 60 Minutes , received honorary citizenship from Saudi Arabia , holds a title from the United Nations , and has gone on a date with actor Will Smith . While Sophia undoubtedly highlights many technological advancements, few surpass Hanson’s achievements in marketing. If Sophia truly were a person, we would acknowledge its role as an influencer .
However, worse than robots or AI being sociopathic agents — goal-oriented without morality or human judgment — these technologies become tools of mass influence for whichever organization or individual controls them.
If you thought the Cambridge Analytica scandal was bad, imagine what Facebook’s algorithms of influence could do if they had an accompanying, human-like face. Or a thousand faces. Or a million. The true value of a persuasive technology is not in its cold, calculated efficiency, but its scale.
Seeing through intent
Recent scandals and exposures in the tech world have left many of us feeling helpless against these corporate giants. Fortunately, many of these issues can be solved through transparency .
There are fundamental questions that are important for social technologies to answer because we would expect the same answers when interacting with another person, albeit often implicitly. Who owns or sets the mandate of this technology? What are its objectives? What approaches can it use? What data can it access?
Since robots could have the potential to soon leverage superhuman capabilities , enacting the will of an unseen owner, and without showing verbal or non-verbal cues that shed light on their intent, we must demand that these types of questions be answered explicitly.
As a roboticist, I get asked the question, “When will robots take over the world?” so often that I’ve developed a stock answer: “As soon as I tell them to.” However, my joke is underpinned by an important lesson: don’t scapegoat machines for decisions made by humans.
I consider myself a robot sympathizer because I think robots get unfairly blamed for many human decisions and errors. It is important that we periodically remind ourselves that a robot is not your friend, your enemy, or anything in between. A robot is a tool, wielded by a person (however far removed), and increasingly used to influence us.
Article by Shane Saunderson , Ph.D. Candidate, Robotics, University of Toronto
This article is republished from The Conversation under a Creative Commons license. Read the original article .
How ‘less-than-one-shot learning’ could open up new venues for machine learning research
If I told you to imagine something between a horse and a bird—say, a flying horse—would you need to see a concrete example? Such a creature does not exist, but nothing prevents us from using our imagination to create one: the Pegasus.
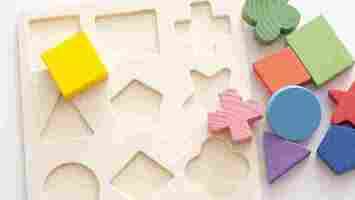
The human mind has all kinds of mechanisms to create new concepts by combining abstract and concrete knowledge it has of the real world. We can imagine existing things that we might have never seen (a horse with a long neck — a giraffe), as well as things that do not exist in real life (a winged serpent that breathes fire — a dragon). This cognitive flexibility allows us to learn new things with few and sometimes no new examples.
In contrast, machine learning and deep learning , the current leading fields of artificial intelligence, are known to require many examples to learn new tasks, even when they are related to things they already know.
Overcoming this challenge has led to a host of research work and innovation in machine learning. And although we are still far from creating artificial intelligence that can replicate the brain’s capacity for understanding , the progress in the field is remarkable.
For instance, transfer learning is a technique that enables developers to finetune an artificial neural network for a new task without the need for many training examples. Few-shot and one-shot learning enable a machine learning model trained on one task to perform a related task with a single or very few new examples. For instance, if you have an image classifier trained to detect volleyballs and soccer balls, you can use one-shot learning to add basketball to the list of classes it can detect.
A new technique dubbed “less-than-one-shot learning” (or LO-shot learning), recently developed by AI scientists at the University of Waterloo, takes one-shot learning to the next level. The idea behind LO-shot learning is that to train a machine learning model to detect M classes, you need less than one sample per class. The technique, introduced in a paper published in the arXiv preprocessor , is still in its early stages but shows promise and can be useful in various scenarios where there is not enough data or too many classes.
The k-NN classifier
The LO-shot learning technique proposed by the researchers applies to the “k-nearest neighbors” machine learning algorithm. K-NN can be used for both classification (determining the category of an input) or regression (predicting the outcome of an input) tasks. But for the sake of this discussion, we’ll still to classification.
As the name implies, k-NN classifies input data by comparing it to its k nearest neighbors ( k is an adjustable parameter). Say you want to create a k-NN machine learning model that classifies hand-written digits. First you provide it with a set of labeled images of digits. Then, when you provide the model with a new, unlabeled image, it will determine its class by looking at its nearest neighbors.
For instance, if you set k to 5, the machine learning model will find the five most similar digit photos for each new input. If, say three of them belong to the class “7,” it will classify the image as the digit seven.
k-NN is an “instance-based” machine learning algorithm. As you provide it with more labeled examples of each class, its accuracy improves but its performance degrades, because each new sample adds new comparisons operations.
In their LO-shot learning paper, the researchers showed that you can achieve accurate results with k-NN while providing fewer examples than there are classes. “We propose ‘less than one’-shot learning (LO-shot learning), a setting where a model must learn N new classes given only M < N examples, less than one example per class,” the AI researchers write. “At first glance, this appears to be an impossible task, but we both theoretically and empirically demonstrate feasibility.”
Machine learning with less than one example per class
The classic k-NN algorithm provides “hard labels,” which means for every input, it provides exactly one class to which it belongs. Soft labels, on the other hand, provide the probability that an input belongs to each of the output classes (e.g., there’s a 20% chance it’s a “2”, 70% chance it’s a “5,” and a 10% chance it’s a “3”).
In their work, the AI researchers at the University of Waterloo explored whether they could use soft labels to generalize the capabilities of the k-NN algorithm. The proposition of LO-shot learning is that soft label prototypes should allow the machine learning model to classify N classes with less than N labeled instances.
The technique builds on previous work the researchers had done on soft labels and data distillation . “Dataset distillation is a process for producing small synthetic datasets that train models to the same accuracy as training them on the full training set,” Ilia Sucholutsky, co-author of the paper, told TechTalks . “Before soft labels, dataset distillation was able to represent datasets like MNIST using as few as one example per class. I realized that adding soft labels meant I could actually represent MNIST using less than one example per class.”
MNIST is a database of images of handwritten digits often used in training and testing machine learning models. Sucholutsky and his colleague Matthias Schonlau managed to achieve above-90 percent accuracy on MNIST with just five synthetic examples on the convolutional neural network LeNet.
“That result really surprised me, and it’s what got me thinking more broadly about this LO-shot learning setting,” Sucholutsky said.
Basically, LO-shot uses soft labels to create new classes by partitioning the space between existing classes.
In the example above, there are two instances to tune the machine learning model (shown with black dots). A classic k-NN algorithm would split the space between the two dots between the two classes. But the “soft-label prototype k-NN” (SLaPkNN) algorithm, as the OL-shot learning model is called, creates a new space between the two classes (the green area), which represents a new label (think horse with wings). Here we have achieved N classes with N-1 samples.
In the paper, the researchers show that LO-shot learning can be scaled up to detect 3N-2 classes using N labels and even beyond.
In their experiments, Sucholutsky and Schonlau found that with the right configurations for the soft labels, LO-shot machine learning can provide reliable results even when you have noisy data.
“I think LO-shot learning can be made to work from other sources of information as well—similar to how many zero-shot learning methods do—but soft labels are the most straightforward approach,” Sucholutsky said, adding that there are already several methods that can find the right soft labels for LO-shot machine learning.
While the paper displays the power of LO-shot learning with the k-NN classifier, Sucholutsky says the technique applies to other machine learning algorithms as well. “The analysis in the paper focuses specifically on k-NN just because it’s easier to analyze, but it should work for any classification model that can make use of soft labels,” Sucholutsky said. The researchers will soon release a more comprehensive paper that shows the application of LO-shot learning to deep learning models.
New venues for machine learning research
“For instance-based algorithms like k-NN, the efficiency improvement of LO-shot learning is quite large, especially for datasets with a large number of classes,” Susholutsky said. “More broadly, LO-shot learning is useful in any kind of setting where a classification algorithm is applied to a dataset with a large number of classes, especially if there are few, or no, examples available for some classes. Basically, most settings where zero-shot learning or few-shot learning are useful, LO-shot learning can also be useful.”
For instance, a computer vision system that must identify thousands of objects from images and video frames can benefit from this machine learning technique, especially if there are no examples available for some of the objects. Another application would be to tasks that naturally have soft-label information, like natural language processing systems that perform sentiment analysis (e.g., a sentence can be both sad and angry simultaneously).
In their paper, the researchers describe “less than one”-shot learning as “a viable new direction in machine learning research.”
“We believe that creating a soft-label prototype generation algorithm that specifically optimizes prototypes for LO-shot learning is an important next step in exploring this area,” they write.
“Soft labels have been explored in several settings before. What’s new here is the extreme setting in which we explore them,” Susholutsky said. “I think it just wasn’t a directly obvious idea that there is another regime hiding between one-shot and zero-shot learning.”
This article was originally published by Ben Dickson on TechTalks , a publication that examines trends in technology, how they affect the way we live and do business, and the problems they solve. But we also discuss the evil side of technology, the darker implications of new tech and what we need to look out for. You can read the original article here .