WhatsApp fined $267M for breaking EU data privacy rules
Did you know Neural is taking the stage this fall ? Together with an amazing line-up of experts, we will explore the future of AI during TNW Conference 2021. Secure your online ticket now !
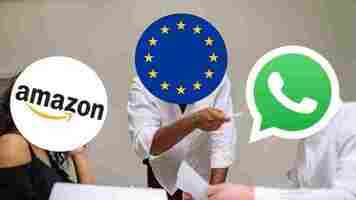
The EU’s data watchdogs are starting to flex their muscles. Weeks after Amazon was fined a record €746 (886M) for breaching GDPR rules, WhatsApp has been hit with the regime’s second-highest penalty.
The €225m ($267m) fine was issued by the Irish Data Protection Commission (DPC). The body is Europe’s l ead authority for WhatsApp owner Facebook, which has its EU headquarters in Ireland.
The DPC said that WhatsApp had infringed the EU’s transparency rules :
The watchdog also ordered the company to take “remedial actions” to ensure its data processing complies with the rules. WhatsApp said it would appeal the “entirely disproportionate” fine.
Regardless of the final decision, the EU may finally be turning its tough talk on data protection into action.
The bloc passed the landmark General Data Protection Regulation ( GDPR ) back in 2018, but critics say it’s been a blunt sword thus far.
Slapping Amazon and WhatsApp with big GDPR fines suggests it’s getting sharper.
Greetings Humanoids! Did you know we have a newsletter all about AI? You can subscribe to it right here .
Google’s new deep learning system could help radiologists analyze X-rays
Did you know Neural is taking the stage this fall ? Together with an amazing line-up of experts, we will explore the future of AI during TNW Conference 2021. Secure your ticket now !
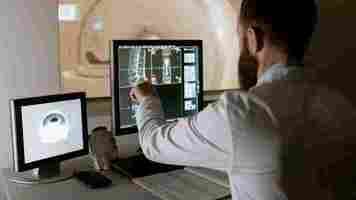
Deep learning can detect abnormal chest x-rays with accuracy that matches that of professional radiologists, according to a new paper by a team of AI researchers at Google published in the peer-reviewed science journal Nature.
The deep learning system can help radiologists prioritize chest x-rays, and it can also serve as a first response tool in emergency settings where experienced radiologists are not available. The findings show that, while deep learning is not close to replacing radiologists, it can help boost their productivity at a time that the world is facing a severe shortage of medical experts.
The paper also shows how far the AI research community has come to build processes that can reduce the risks of deep learning models and create work that can be further built on in the future.
Searching for abnormal chest x-rays
The advances in AI-powered medical imaging analysis are undeniable. There are now dozens of deep learning systems for medical imaging that have received official approval from FDA and other regulatory bodies across the world.
But the problem with most of these models is that they have been trained for a very narrow task, such as finding traces of a specific disease and conditions in x-ray images. Therefore, they will only be useful in cases where the radiologist knows what to look for.
But radiologists don’t necessarily start by looking for a specific disease. And building a system that can detect every possible disease is extremely difficult “if not impossible.
“[The] wide range of possible CXR [chest x-rays] abnormalities makes it impractical to detect every possible condition by building multiple separate systems, each of which detects one or more pre-specified conditions,” Google’s AI researchers write in their paper.
Their solution was to create a deep learning system that detects whether a chest scan is normal or contains clinically actionable findings. Defining the problem domain for deep learning systems is an act of finding the balance between specificity and generalizability. On one end of the spectrum are deep learning models that can perform very narrow tasks (e.g., detecting pneumonia or fractures) at the cost of not generalizing to other tasks (e.g., detecting tuberculosis). And on the other end are systems that answer a more general question (e.g., is this x-ray scan normal or does it need further examination?) but can’t solve more specific problems.
The intuition of Google’s researchers was that abnormality detection can have a great impact on the work of radiologists, even if the trained model didn’t point out specific diseases.
“A reliable AI system for distinguishing normal CXRs from abnormal ones can contribute to prompt patient workup and management,” the researchers write.
For example, such a system can help de-prioritize or exclude cases that are normal, which can speed up the clinical process.
Although the Google researchers did not provide precise details of the model they used, the paper mentions EfficientNet, a family of convolutional neural networks (CNN) that are renowned for achieving state-of-the-art accuracy on computer vision tasks at a fraction of the computational costs of other models.
B7, the model used for the x-ray abnormality detection, is the largest of the EfficientNet family and is composed of 813 layers and 66 million parameters (though the researchers probably adjusted the architecture based on their application). Interestingly, the researchers did not use Google’s TPU processors and used 10 Tesla V100 GPUs to train the model.
Avoiding unnecessary bias in the deep learning model
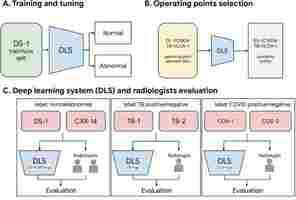
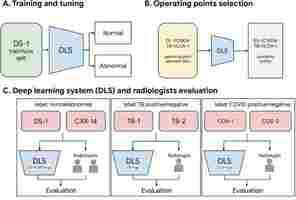
Perhaps the most interesting part of Google’s project is the intensive work that was done to prepare the training and test dataset. Deep learning engineers are often faced with the challenge of their models picking up the wrong biases hidden in their training data. For example, in one case, a deep learning system for skin cancer detection had mistakenly learned to detect the presence of ruler marks on skin. In other cases, models can become sensitive to irrelevant factors, such as the brand of equipment used to capture the images. And more importantly, it is important that a trained model can maintain its accuracy across different populations.
To make sure problematic biases didn’t creep into the model, the researchers used six independent datasets for training and test.
The deep learning model was trained on more than 250,000 x-ray scans originating from five hospitals in India. The examples were labeled as “normal” or “abnormal” based on information extracted from the outcome report.
The model was then evaluated with new chest x-rays obtained from hospitals in India, China, and the U.S. to make sure it generalized to different regions.
The test data also contained x-ray scans for two diseases that were not included in the training dataset, TB and Covid-19, to check how the model would perform on unseen diseases.
The accuracy of the labels in the dataset were independently reviewed and confirmed by three radiologists.
The researchers have made the labels publicly available to help future research on deep learning models for radiology. “To facilitate the continued development of AI models for chest radiography, we are releasing our abnormal versus normal labels from 3 radiologists (2430 labels on 810 images) for the publicly-available CXR-14 test set. We believe this will be useful for future work because label quality is of paramount importance for any AI study in healthcare,” the researchers write.
Augmenting radiologist with deep learning
Radiology has had a rocky history with deep learning.
In 2016, deep learning pioneer Geoffrey Hinton said, “I think if you work as a radiologist, you’re like the coyote that’s already over the edge of the cliff but hasn’t yet looked down, so it doesn’t yet realize there’s no ground underneath him. People should stop training radiologists now. It’s just completely obvious that within five years, deep learning is going to do better than radiologists because it’s going to get a lot more experience “it might be ten years, but we’ve got plenty of radiologists already.”
But five years later, AI is not anywhere close to driving radiologists out of their jobs. In fact, there’s still a severe shortage of radiologists across the globe, even though the number of radiologists has increased. And a radiologist’s job involves a lot more than looking at x-ray scans .
In their paper, the Google researchers note that their deep learning model succeeded in detecting abnormal x-ray with accuracy that is comparable and in some cases superior to human radiologists. However, they also point out that the real benefit of this system is when it is used to improve the productivity of radiologists.
To evaluate the efficiency of the deep learning system, the researchers tested it in two simulated scenarios, where the model assisted a radiologist by either helping prioritize scans that were found to be abnormal or excluding scans that were found to be normal. In both cases, the combination of deep learning and radiologist resulted in a significant improvement to the turnaround time.
“Whether deployed in a relatively healthy outpatient practice or in the midst of an unusually busy inpatient or outpatient setting, such a system could help prioritize abnormal CXRs for expedited radiologist interpretation,” the researchers write.
How to shift your AI focus from accuracy to value
“All models are wrong, but some are useful.” This is a famous quote from 20th century statistical thinker, George E.P. Box.
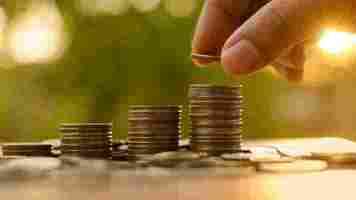
This might seem like a strange message- shouldn’t all the models we build be as correct as possible? However, as a data scientist myself, I see great wisdom in this statement. After all, businesses don’t buy AI for model accuracy, they buy it to drive business value. Many businesses are investing in AI today without fully realizing its potential to deliver business impact. It’s time to shift the conversation.
The problem? Most groups embark on building their AI solution by discussing what they want to predict, and quickly shift to a discussion about model accuracy. This strategy often leads data scientists into the doldrums of model metrics that have no connection to business KPIs. Instead, we must focus on desired business outcomes, and what actions AI can prescribe we take in order to achieve those goals.
Let’s illustrate this with an example of a software company. The accounts receivable team of this company might use AI to predict if an invoice will be paid on time. In isolation this prediction has limited business value — an accurate prediction of each customer paying on time doesn’t quite meet the goal of shrinking the cash revenue cycle. Instead, this team should think about the AI solution holistically: how can they align their prediction with key recommendations and actions that will help the user focus their time.
So how do we achieve this? We need to break down the silos between business leaders and data scientists. Critically, let’s get business leaders and data scientists to follow four key pillars together, which will align organizations around a smarter, core approach:
MEASURE the KPI. What is the business outcome we are tracking and used as the measure to track impact for your model?
INTERVENE based on what the AI prescribes. What organizational levers and limitations exist and how can your AI provide guidance?
EXPERIMENT to measure impact. Build models and deploy these in controlled experiments to attribute impact to the use of AI.
ITERATE by constantly monitoring, optimizing and experimenting. Data changes, opportunities arise, no model lives in perpetuity.
These four pillars will help data scientists surface more valuable questions to their business counterparts and give said business leaders a deeper understanding of the power of AI within the organization. Too often it’s difficult or time consuming for technologists to educate their business counterparts on AI or ask them why a particular predictive model is being suggested. AI can be more than the datasets that power it. Adopting these four pillars and having honest conversations early and often can lead to more agility and resilience — critical as local to global events shift the business landscape around us, from temporary anomalies to black swan events.
Let’s return to the business outcome we were discussing – receiving payment on invoices.
Typically, businesses will build a predictive model to flag which customers may be at risk of not paying on time. But if we focus on a better way of measuring impact, we’d turn that predictive flag into a prescriptive solution and train the model to increase the expected revenue received within 30 days of sending the invoice.
Today, the staff in accounts receivable may have several tools at their disposal to ensure that payment is collected within 30 days. Each have their own effectiveness, from phone calls to email nudges, automatic payment suggestions or texts about suspending service. Staff can choose from any number of these actions in order to try to hit a target, however they may be constrained on where to spend their time. A model that predicts outcomes alone fall short of helping the staff choose what action to take. Instead, try building models that predict outcomes given those interventions, therefore influencing actions that yield optimal results.
We’ve now turned our predictive flag program into prescribed interventions. Models are not meant to be static, however, and so running tests, tracking real-time interactions, getting access to temporal data (in sequence) and monitoring your KPI is critical step to making sure your models don’t crumble when facing unforeseen events. Models will not live in perpetuity, so be agile and know how to deploy new models. Iteration is not only about fixing problem; it is also about opportunity. Yes, it will let you respond quickly to problems like data drift, but it also lets you experiment – constantly moving your business forward.
This mind shift from predictive to prescriptive is a natural evolution in how we understand and harness AI within their business. And it is more important in today’s highly-unpredictable economic and competitive business climate, where the ability to make real-time decisions and quickly deliver value can separate the winners from the losers.