Why people should get paid to let AI do their jobs for them
The idea of a universal minimum/basic income (UBI) isn’t new or nearly as radical as those both in favor and opposed to it would have you believe. Dozens of cities across the globe are either currently running or have run UBI test programs. And the results are usually positive.
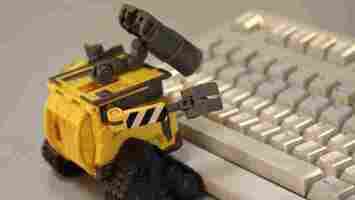
Simply put: the outcomes for people who receive a UBI are typically demonstrably better than people in similar financial and economic situations who don’t.
But a significant number of people ranging from laypersons to economics experts believe that paying people for what they consider “not working” is a bad idea.
The solution, of course, is artificial intelligence. What if we scrapped the idea of a UBI and, instead, allowed AI to do all the work while we just cash the paychecks?
What? This is also not a new or radical idea. Workplace automation is occurring across most employment domains already. Whether you’re in danger of being displaced by automation, you’ve become a cyborg by adding machine learning solutions to your normal working routine, or you just save 30 seconds per email by clicking on Google’s automated response suggestions, you probably use AI to do your day-to-job. Even if you don’t, the writing is on the wall.
In the near future, it’ll be more common for general industries to look for automated solutions first before creating employment opportunities for humans.
Background: The conversation about UBI used to be entirely centered on caring for the most vulnerable segments of society. Especially in the wake of COVID-19 where unemployment and houselessness are ravaging even previously-stable economic areas.
But we won’t know the full extent of the effects of the pandemic until it passes entirely. Whether that occurs in a matter of months or we’ve still got years to go, the end result will almost certainly involve a massive global industrial and commercial shift.
Some of those jobs that got put on hold when the world shut down will go away entirely, others may change in ways we cannot predict.
A couple of years ago most of us couldn’t have imagined such a large portion of the workforce pivoting to work from home. Now, we’ve given thousands of businesses an opportunity to consider what a people-free workplace might look like. It’s not outlandish to imagine a paradigm shift towards employeeless businesses that maximize profits by limiting overhead and cutting human-related costs.
Capitalism
The current paradigm is simple: you work, you make money, you pay taxes, those taxes get spread around to do stuff for everyone… including people who don’t work or pay taxes. Some people think this is fine because of the greater good , others feel like they shouldn’t have to work so others don’t have to.
It might be easier to just scrap the idea of a UBI and, instead, give us all AI employment avatars so we don’t have to work but still collect a regular paycheck. After all, this is exactly what rich people do, but instead of creating an AI that does something useful they just accumulate wealth instead.
The roundabout average interest on a million dollars in a bank account is usually somewhere around $30K a year . The US government pegs the minimum wage at much less than that . Which means, according to Uncle Sam, you should be able to live on less than the interest from a seven-figure trust fund.
Yet our government, society, and the capitalist culture all seem to agree that getting paid for doing nothing is wrong unless you’re wealthy. And that’s probably a good thing. Because the government really needs that money to accumulate in banks so it and the banks can borrow it without asking (that’s how the interest gets there).
But the undeniable fact of the matter is that someone making the minimum wage, and contributing directly to the workforce, earns less than someone who cashes in a million dollar trust fund and just doesn’t feel like working.
A non-political view on this would be: that if it’s okay for us to let our accumulated wealth generate income for us, it should be universally acceptable for us to design AI systems that do our job for us.
Unfortunately, we all know that isn’t the case. In 2016 a Redditor (who’s since deleted their post and account, thus we won’t name them here) posted their account of allegedly being fired from a tech job after six years without actually doing any work. The clever (or lazy, depending on your view) employee, according to the post, designed a program to automate their coding duties and just borked off at work all day for six whole years until they got caught.
In the real world: That example might confirm everyone’s fears that society might collapse if we just give people money and take away their will to work, grow, and succeed. But, we’re only guessing that everyone who automates their job will want to take a permanent vacation.
The fear that someone who doesn’t deserve it will get something for free that we’ve worked so hard for is often a strong motivator against altruistic ideas such as UBI.
But there’s no system that will take care of only those who need it while remaining impervious to human corruption or laziness.
The reality is that we know beyond a shadow of the doubt a UBI could save lives. There are people who won’t eat today who otherwise would if they had money.
But a guaranteed income from a government ran by politicians owned by corporate lobbyists, as things are in the US and other capitalist regimes, may not be the best way to help We The People, whether you support UBI or not.
Instead, let’s take the aforementioned Redditor who automated their job as an example. What if, instead of punishing that person by firing them, we used them as a prototype for an “automation employment avatar?”
How it would work: Let’s stick to the US for an example, as it’s a country mired in the kind of partisanship that prevents UBI from being seriously discussed. Based on what conservatives and liberals have said about UBI, the only thing we all agree on is that US citizens who are not incarcerated or otherwise prevented from the full rights of citizenship should have the right to work.
So, instead of giving citizens a UBI, the government could give humans the explicit right to employment. With the right to employment, we could legislate huge tax benefits for corporations that employ humans. And simply get rid of tax breaks for those that don’t.
Those companies that automate existing human positions or that feature automation beyond a certain threshold, would be ineligible for tax breaks unless they paid out “automation avatar” salaries to humans in order to compensate for worker displacement.
In this way, companies like Amazon that manage to avoid paying taxes would still have to contribute to society in the same way that a mom and pop pizza restaurant does when it’s forced to pay its full share.
If that pizza place wants to lay off its cook in favor of a robot oven, it’ll have to pay that human a continuing salary if it wants to participate in the US tax credit system. For new businesses, say an automated pizza kitchen opens up on the same block as our mom and pop human-run shop, it’ll have to pay human salaries too, if its owners want tax breaks, even if they don’t employ any real people.
And, if the same went for Amazon, we could likely solve poverty in the US faster than you can say “two-day shipping.”
It’s not a perfect plan, but neither is UBI. And the status quo is about as anti-human as it gets. There are at least 18.6 million people in the US who could live above the poverty line off the annual interest from their bank accounts alone, but more than 40 million people in the US currently exist in poverty.
Greetings Humanoids! Did you know we have a newsletter all about AI? You can subscribe to it right here .
MIT’s coronavirus-killer robot uses UV-C light to disinfect warehouses
A new MIT robot that uses UV light to kill the coronavirus could be used to disinfect warehouses, schools, and offices.
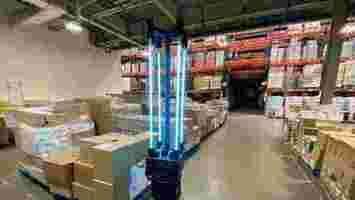
The system has already been used to sanitize the Greater Boston Food Bank. In tests, the robot covered a 4,000 square foot area of the warehouse within 30 minutes, providing enough light to neutralize around 90% of coronavirus particles.
Scientists from MIT‘s Computer Science and Artificial Intelligence Lab (CSAIL) designed the light-emitting vertical bars that shine UV light onto surfaces. They then attached the fixture to a mobile robotic base produced by Ava Robotics.
You can check it out in action in the video below:
The researchers believe the system could be used in an array of different venues, such as factories, restaurants, and supermarkets. But first, they need to enhance its autonomy.
Increasing autonomy
The system uses a short-wavelength of ultraviolet light, known as UV-C, to break apart germ DNA. Studies show that UV-C can kill other coronaviruses, such as SARS, and it’s already widely used to eradicate COVID-19 in China . However, it can also damage skin and eye cells, so humans need to wait outside while the lights pump out their ghostly blue beams.
Currently, teleoperators must first teach the robot a route around the site. It then follows waypoints around a map of the venue, which show the droid where to go and point the light.
The next step is enabling the robot to adapt to changes in its environment. In the food bank, the position of pallets varies every day as staff move them to different aisles in the warehouse.
“We’ve started to identify known static components of the map,” CSAIL research scientist Alyssa Pierson told TNW. “These are things that never change, such as the walls in the environment as well as large metal shelving for the pallets.
“We’re also looking at defining zones where things change so there may be obstacles there, which allows the robot to focus on where its attention should be.”
The system currently uses a 3D camera to navigate around obstacles and 2D LiDARS to measure distances by illuminating targets with light. Pearson says this is sufficient for warehouses, but that further sensors may be required for other locations.
But for now, her team is focused on food banks, which have faced a huge rise in demand since the pandemic began. Protecting their staff from infection will help them support the millions of people pushed into food insecurity .
A beginner’s guide to the math that powers machine learning
How much math knowledge do you need for machine learning and deep learning? Some people say not much. Others say a lot. Both are correct, depending on what you want to achieve.
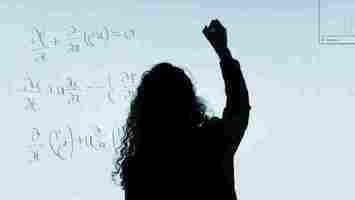
There are plenty of programming libraries, code snippets, and pretrained models that can get help you integrate machine learning into your applications without having a deep knowledge of the underlying math functions.
But there’s no escaping the mathematical foundations of machine learning . At some point in your exploration and mastering of artificial intelligence, you’ll need to come to terms with the lengthy and complicated equations that adorn AI whitepapers and machine learning textbooks.
In this post, I will introduce some of my favorite machine learning math resources. And while I don’t expect you to have fun with machine learning math, I will also try my best to give you some guidelines on how to make the journey a bit more pleasant.
Start with the basics
Many machine learning books tell you that having a working knowledge of linear algebra. I would argue that you need a lot more than that. Extensive experience with linear algebra is a must-have—machine learning algorithms squeeze every last bit out of vector spaces and matrix mathematics.
You also need to know a good bit of statistics and probability, as well as differential and integral calculus, especially if you want to become more involved in deep learning .
There are plenty of good textbooks, online courses, and blogs that explore these topics. But my personal favorite is Khan Academy’s math courses . Sal Khan has done a great job of putting together a comprehensive collection of videos that explain different math topics. And it’s free, which makes it even better.
Although each of the videos (which are also available on YouTube) explain a separate topic, going through the courses end-to-end provides a much richer experience.
I recommend the linear algebra course in particular. Here, you’ll find everything you need about vector spaces, linear transformations, matrix transformations, and coordinate systems. The course has not been tailored for machine learning, and many of the examples are about 2D and 3D graphic systems, which are much easier to visualize than the multidimensional spaces of machine learning problems. But they discuss the same concepts you’ll encounter in machine learning books and whitepapers. In the course are some hidden gems like least square calculations and eigenvectors, which are important topics in machine learning.
The calculus course are a bit more fragmented, but it might be a good feature for readers who already have a strong foundation and just want to brush up their skills. Khan includes precalculus, differential calculus, and integral calculus courses that cover the foundations. The multivariable calculus course discusses some of the topics that are central to deep learning, such as gradient descent and partial derivatives.
There are also several statistics courses in Khan Academy’s platform, and there are some overlaps between them. They all discuss some of the key concepts you need in data science and machine learning, such as random variables, distributions, confidence intervals, and the difference between continuous and categorical data. I recommend the college statistics course , which includes some extra material that is relevant to machine learning, such as the Bayes theorem.
To be clear, Khan Academy’s courses are not a replacement for the math textbook and classroom. They are not very rich in exercises. But they are very rich in examples, and for someone who just needs to blow the dust off their algebra knowledge, they’re great. Sal talks very slowly, probably to make the videos usable for a wider audience who are not native English speakers. I run the videos on 1.5x speed and have no problem understanding them, so don’t let the video lengths taunt you.
Specialized books and courses on machine learning math
Vanilla algebra and calculus are not enough to get comfortable with the mathematics of machine learning. Machine learning concepts such as loss functions, learning rate, activation functions, and dimensionality reduction are not covered in classic math books. There are more specialized resources for that.
My favorite is Mathematics for Machine Learning . Written by three AI researchers, the provides you with a strong foundation to explore the workings of different components of machine learning algorithms.
The book is split into two parts. The first part is mathematical foundations, which is basically a revision of key linear algebra and calculus concepts. The authors cover a lot of material in little more than 200 pages, so most of it is skimmed over with one or two examples. If you have a strong foundation, this part will be a pleasant read. If you find it hard to grasp, you can combine the chapters with select videos from Khan’s YouTube channel. It’ll become much easier.
The second part of the book focuses on machine learning mathematics. You’ll get into topics such as regression, dimensionality reduction, support vector machines, and more. There’s no discussion of artificial neural networks and deep learning concepts, but being focused on the basics makes this book a very good introduction to the mathematics of machine learning.
As the authors write on their website: “The book is not intended to cover advanced machine learning techniques because there are already plenty of books doing this. Instead, we aim to provide the necessary mathematical skills to read those other books.”
For a more advanced take on deep learning, I recommend Hands-on Mathematics for Deep Learning . This book also contains an intro on linear algebra, calculus, and probability and statistics. Again, this section is for people who just want to jar their memory. It’s not a basic introductory book.
The real value of this book comes in the second section, where you go into the mathematics of multilayer perceptrons, convolutional neural networks (CNN), and recurrent neural networks (RNN). The book also goes into the logic of other crucial concepts such as regularization (L1 and L2 norm), dropout layers, and more.
These are concepts that you’ll encounter in most books on machine learning and deep learning. But knowing the mathematical foundations will help you better understand the role hyperparameters play in improving the performance of your machine learning models.
A bonus section dives into advanced deep learning concepts, such as the attention mechanism that has made Transformers so efficient and popular, generative models such as autoencoders and generative adversarial networks , and the mathematics of transfer learning .
When should you learn machine learning mathematics?
Agreeably, mathematics is not the most fun way to start machine learning education, especially if you’re self-learning. Fortunately, as I said at the beginning of this article, you don’t need to begin your machine learning education by poring over double integrals, partial derivatives, and mathematical equations that span a page’s width.
You can start with some of the more practical resources on data science and machine learning. A good introductory book is Principles of Data Science , which gives you a good overview of data science and machine learning fundamentals along with hands-on coding examples in Python and light mathematics. Hands-on Machine Learning and Python Machine Learning are two other books that are a little more advanced and also give deeper coverage of the mathematical concepts. Udemy’s Machine Learning A-Z is an online course that combines coding with visualization in a very intuitive way.
I would recommend starting with one or two of the above-mentioned books and courses. They will give you a working knowledge of the basics of machine learning and deep learning and prepare your mind for the mathematical foundations. Once you know have a solid grasp of different machine learning algorithms, learning the mathematical foundations becomes much more pleasant.
As you master the mathematics of machine learning, you will find it easier to find new ways to optimize your models and tweak them for better performance. You’ll also be able to read the latest cutting edge papers that explain the latest findings and techniques in deep learning, and you’ll be able to integrate them into your applications. In my experience, the mathematics of machine learning is an ongoing educational experience. Always look for new ways to hone your skills.
This article was originally published by Ben Dickson on TechTalks , a publication that examines trends in technology, how they affect the way we live and do business, and the problems they solve. But we also discuss the evil side of technology, the darker implications of new tech and what we need to look out for. You can read the original article here .