Why we should be excited – and worried – about Neuralink’s brain-computer interface
Some weeks ago, a nine-year-old macaque monkey called Pager successfully played a game of Pong with its mind.
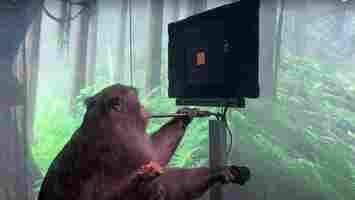
While it may sound like science fiction, the demonstration by Elon Musk’s neurotechnology company Neuralink is an example of a brain-machine interface in action (and has been done before ).
A coin-sized disc called a “ Link ” was implanted by a precision surgical robot into Pager’s brain, connecting thousands of micro threads from the chip to neurons responsible for controlling motion.
Brain-machine interfaces could bring tremendous benefit to humanity. But to enjoy the benefits, we’ll need to manage the risks down to an acceptable level.
A perplexing game of Pong
Pager was first shown how to play Pong in the conventional way, using a joystick . When he made a correct move, he’d receive a sip of banana smoothie. As he played, the Neuralink implant recorded the patterns of electrical activity in his brain. This identified which neurons controlled which movements.
The joystick could then be disconnected, after which Pager played the game using only his mind — doing so like a boss.
This Neuralink demo built on an earlier one from 2020, which involved Gertrude the Pig. Gertrude had the Link installed and output recorded, but no specific task was assessed.
Read more: Neuralink put a chip in Gertrude the pig’s brain. It might be useful one day
Helping people with brain injury
According to Neuralink, its technology could help people who are paralysed with spinal or brain injuries, by giving them the ability to control computerized devices with their minds. This would provide paraplegics, quadriplegics and stroke victims the liberating experience of doing things by themselves again.
Prosthetic limbs might also be controlled by signals from the Link chip. And the technology would be able to send signals back, making a prosthetic limb feel real .
Cochlear implants already do this, converting external acoustic signals into neuronal information, which the brain translates into sound for the wearer to “hear”.
Neuralink has also claimed its technology could remedy depression, addiction , blindness, deafness and a range of other neurological disorders. This would be done by using the implant to stimulate areas of the brain associated with these conditions.
A game-changer
Brain-machine interfaces could also have applications beyond the therapeutic . For a start, they could offer a much faster way of interacting with computers, compared to methods that involve using hands or voice.
A user could type a message at the speed of thought and not be limited by thumb dexterity. They’d only have to think the message and the implant could convert it to text. The text could then be played through software that converts it to speech.
Perhaps more exciting is a brain-machine interface’s ability to connect brains to the cloud and all its resources. In theory, a person’s own “native” intelligence could then be augmented on demand by accessing cloud-based artificial intelligence (AI).
Human intelligence could be greatly multiplied by this. Consider for a moment if two or more people wirelessly connected their implants. This would facilitate a high-bandwidth exchange of images and ideas from one to the other.
In doing so they could potentially exchange more information in a few seconds than would take minutes, or hours, to convey verbally.
But some experts remain skeptical about how well the technology will work, once it’s applied to humans for more complex tasks than a game of Pong. Regarding Neuralink, Anna Wexler, a professor of medical ethics and health policy at the University of Pennsylvania, said:
Can Neuralink be hacked?
At the same time, concerns about such technology’s potential harm continue to occupy brain-machine interface researchers.
Without bulletproof security, it’s possible hackers could access implanted chips and cause a malfunction or misdirection of its actions. The consequences could be fatal for the victim.
Some may worry powerful artificial AI working through a brain-machine interface could overwhelm and take control of the host brain.
The AI could then impose a master-slave relationship and, the next thing you know, humans could become an army of drones. Elon Musk himself is on record saying artificial intelligence poses an existential threat to humanity.
He says humans will need to eventually merge with AI, to remove the “existential threat” advanced AI could present:
Musk has famously compared AI research and development with “summoning the demon”. But what can we reasonably make of this statement? It could be interpreted as an attempt to scare the public and, in so doing, pressure governments to legislate strict controls over AI development.
Musk himself has had to negotiate government regulations governing the operations of autonomous and aerial vehicles such as his SpaceX rockets.
Hasten slowly
The crucial challenge with any potentially volatile technology is to devote enough time and effort into building safeguards. We’ve managed to do this for a range of pioneering technologies, including atomic energy and genetic engineering.
Autonomous vehicles are a more recent example. While research has shown the vast majority of road accidents are attributed to driver behaviour, there are still situations in which AI controlling a car won’t know what to do and could cause an accident.
Read more: Are autonomous cars really safer than human drivers?
Years of effort and billions of dollars have gone into making autonomous vehicles safe, but we’re still not quite there. And the travelling public won’t be using autonomous cars until the desired safety levels have been reached. The same standards must apply to brain-machine interface technology.
It is possible to devise reliable security to prevent implants from being hacked. Neuralink (and similar companies such as NextMind and Kernel) have every reason to put in this effort. Public perception aside, they would be unlikely to get government approval without it.
Last year the US Food and Drug Administration granted Neuralink approval for “breakthrough device” testing, in recognition of the technology’s therapeutic potential.
Moving forward, Neuralink’s implants must be easy to repair, replace and remove in the event of malfunction, or if the wearer wants it removed for any reason. There must also be no harm caused, at any point, to the brain.
While brain surgery sounds scary, it has been around for several decades and can be done safely.
When will human trials start?
According to Musk, Neuralink’s human trials are set to begin towards the end of this year. Although details haven’t been released, one would imagine these trials will build on previous progress. Perhaps they will aim to help someone with spinal injuries walk again.
The neuroscience research needed for such a brain-machine interface has been advancing for several decades. What was lacking was an engineering solution that solved some persistent limitations, such as having a wireless connection to the implant, rather than physically connecting with wires.
On the question of whether Neuralink overstates the potential of its technology, one can look to Musk’s record of delivering results in other enterprises (albeit after delays ).
The path seems clear for Neuralink’s therapeutic trials to go ahead. More grandiose predictions, however, should stay on the backburner for now.
A human-AI partnership could have a positive future as long as humans remain in control. The best chess player on Earth is not an AI, nor a human. It’s a human-AI team known as a Centaur .
And this principle extends to every field of human endeavor AI is making inroads into.
This article by David Tuffley , Senior Lecturer in Applied Ethics & CyberSecurity, Griffith University , is republished from The Conversation under a Creative Commons license. Read the original article .
Understand adversarial attacks by doing one yourself with this tool
In recent years, the media have been paying increasing attention to adversarial examples , input data such as images and audio that have been modified to manipulate the behavior of machine learning algorithms . Stickers pasted on stop signs that cause computer vision systems to mistake them for speed limits; glasses that fool facial recognition systems, turtles that get classified as rifles — these are just some of the many adversarial examples that have made the headlines in the past few years.
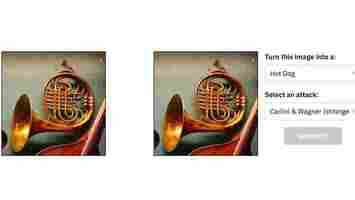
There’s increasing concern about the cybersecurity implications of adversarial examples , especially as machine learning systems continue to become an important component of many applications we use. AI researchers and security experts are engaging in various efforts to educate the public about adversarial attacks and create more robust machine learning systems .
Among these efforts is adversarial.js , an interactive tool that shows how adversarial attacks work. Released on GitHub last week, adversarial.js was developed by Kenny Song, a graduate student at the University of Tokyo doing research on the security of machine learning systems. Song hopes to demystify adversarial attacks and raise awareness about machine learning security through the project.
Crafting your own adversarial examples
Song has designed adversarial.js with simplicity in mind. It is written in Tensorflow.js, the JavaScript version of Google’s famous deep learning framework.
“I wanted to make a lightweight demo that can run on a static webpage. Since everything is loaded as JavaScript on the page, it’s really easy for users to inspect the code and tinker around directly in the browser,” Song told TechTalks .
Song has also launched a demo website that hosts adversarial.js. To craft your own adversarial attack, you choose a target deep learning model and a sample image. You can run the image through the neural network to see how it classifies it before applying the adversarial modifications.
As adversarial.js displays, a well-trained machine learning model can predict the correct label of an image with very high accuracy.
The next phase is to create the adversarial example. The goal here is to modify the image in a way that it doesn’t change to a human observer but causes the targeted machine learning model to change its output.
After you choose a target label and an attack technique and click “Generate,” adversarial.js creates a new version of the image that is slightly modified. Depending on the technique you use, the modifications might be more or less visible to the naked eye. But to the target deep learning model , the difference is tremendous.
In our case, we are trying to fool the neural network into thinking our stop sign is a 120km/hr speed limit sign. Hypothetically, this would mean that a human driver would still stop when seeing the sign, but a self-driving car that uses neural networks to make sense of the world would dangerously speed past it.
Adversarial attacks are not an exact science, and this is one of the things that adversarial.js displays very well. If you tinker with the tool a bit, you’ll see that in many cases, the adversarial techniques do not work consistently. In some cases, the perturbation does not result in the machine learning model changing its output to the desired class but instead causes it to lower its confidence in the main label.
Understanding the threat of adversarial attacks
“I got interested in adversarial examples because they break our fantasy that neural networks have human-level perceptual intelligence,” Song says. “Beyond the immediate problems it brings, I think understanding this failure mode can help us develop more intelligent systems in the future.”
Today, you can run machine learning models in applications running on your computer, phone, home security camera, smart fridge, smart speaker, and many other devices.
Adversarial vulnerabilities make these machine learning systems unstable in different environments . But they can also create security risks that we have yet to understand and deal with.
“Today, there’s a good analogy to the early days of the internet,” Song says. “In the early days, people just focused on building cool applications with new technology, and assumed everyone else had good intentions. We’re in that phase of machine learning now.”
Song warns that bad actors will find ways to take advantage of vulnerable machine learning systems that were designed for a “best-case, I.I.D. [independent and identically distributed], non-adversarial world.” And few people understand the risk landscape, partially due to the knowledge being locked in research literature. In fact, adversarial machine learning has been discussed among AI scientists since the early 2000s and there are already thousands of papers and technical articles on the topic.
“Hopefully, this project can get people thinking about these risks, and motivate investing resources to address them,” Song says.
The machine learning security landscape
“Adversarial examples are just one problem,” Song says, adding that there are more attack vectors like data poisoning , model backdooring , data reconstruction, or model extraction.
There’s growing momentum across different sectors to create tools for improving the security of machine learning systems. In October, researchers from 13 organizations, including Microsoft, IBM, Nvidia, MITRE, released the Adversarial ML Threat Matrix , a framework to help developers and adopters of machine learning technology to identify possible vulnerabilities in their AI systems and patch them before malicious actors exploit them. IBM’s research unit is separately involved in a lot of research on creating AI systems that are robust to adversarial perturbations. And the National Institute of Standards and Technology, the research arm of the U.S. Department of Commerce, has launched TrojAI , an initiative to combat trojan attacks on machine learning systems.
And independent researchers such as Song will continue to make their contributions to this evolving field. “I think there’s an important role for next-gen cybersecurity companies to define best practices in this space,” Song says.
This article was originally published by Ben Dickson on TechTalks , a publication that examines trends in technology, how they affect the way we live and do business, and the problems they solve. But we also discuss the evil side of technology, the darker implications of new tech and what we need to look out for. You can read the original article here .
Scientists use supercomputers and AI to determine how good (or deadly) your street drugs are
A team of researchers from the University of Victoria have developed an AI system capable of determining the expected chemical makeup of drugs. While it involves supercomputers and a robust cocktail of cloud-based machine learning technologies, the ultimate goal is to make it dead-simple for just about anyone to tell what’s in their drugs.
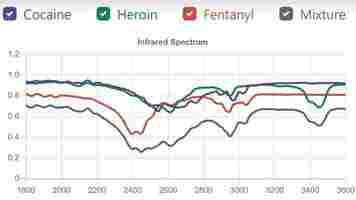
Approximately 70,000 deaths from drug overdose are recorded annually in the US alone. While the causes are both myriad and systemic, a significant number of these tragedies could potentially be avoided if consumers knew what was in their drugs.
The issue relates to those who take both ‘legal’ prescription drugs under the care and advisement of properly-licensed medical professional and those who abuse prescription drugs or use so-called ‘street’ drugs.
[Read next: Meet the 4 scale-ups using data to save the planet ]
According to a report from Ken Strandberg in Technology Networks’ Informatics, the project came about to address concerns over things such as fentanyl levels in opioids and inconsistencies in the unregulated prescription drug markets.
When most laypersons think about drug testing, they’re probably imagining something like a urine sample or a hair test. But drug ‘checking’ is used to determine what’s in a drug itself. Typically, we have to take a drug maker at their word. When big pharma tells us what’s in our pills, we pretty much have to believe it.
And the same goes for the so-called ‘street’ drug market. Without a laboratory and some dedicated equipment it’s virtually impossible for someone to determine what’s actually in the molly, MDMA, or other drugs people are taking.
The big idea here is to ultimately develop a system for medical professionals – such as pharmacists – to quickly and accurately determine what’s actually in the drugs they issue. But the scientists also want to see their work made available to the general public.
Strandberg’s piece quotes Dennis Hore, a member of the University of Victoria team:
Dirty drugs, whether prescription or ‘street,’ are responsible for untold deaths. A system that can quickly and easily determine what’s in your drugs could be an incredible game-changer, but shrinking a laboratory down to kiosk size is no simple task.
Where normal drug analysis involves physical chemistry – with machines and beakers and so forth – the Victoria team’s system relies on a robust cocktail of AI, machine learning, and supercomputers to make ‘surface’ inferences. The reason for this is simple: we can’t put a glass beaker or a centrifuge on the internet, but we can use cloud compute to put supercomputer-based AI online.
Quick take: The researchers are using brute force here, and not just because the supercomputer they’re using (called Arbutus 2) has 1,000s of Intel Xeon processors. The AI powering the analysis uses a kitchen sink approach involving several different types of AI and ML paradigms. This, according to the team, makes it possible for the system to determine both known quantities and completely novel drug compounds.
In much the same way that laboratory testing helps to protect pharmacies and their patients from ‘bad batches’ and needle exchange programs help protect addicts from disease, this AI system could serve as a new, powerful form of protection for drug users.