Why you shouldn’t expect Tesla’s ‘Full Self Driving’ to come out of beta any time soon
Tesla’s recent decision to open its Full Self Driving (FSD) beta to new owners has created quite a splash in both the automobile and consumer tech markets. This is an exciting time to be a Tesla owner, FSD is one of the most innovative software packages we’ve seen in an automobile. But it’s also misleading.
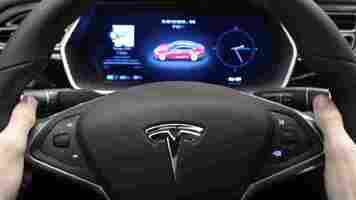
As I’ve written before , Tesla’s Full Self Driving software is not a full self driving system. It only works under certain circumstances to perform specific tasks related to driving: it cannot safely perform an end-to-end traversal that requires it to navigate city streets, highways, and parking lots in unknown territory.
Background
FSD is a beta software in every sense of the term. It’s a strong enough AI system to demonstrate the core concepts and it’s functional enough to make it desirable for consumers. Who doesn’t want to push a button and summon their sports car from a parking lot like Batman?
But you have to assume the risk that your car will damage property or injure people when you use its FSD features – something that’s counter-intuitive to a consumer product market where death is commonly associated with mechanical error.
Most insurance companies that cover vehicles with autonomous capabilities consider the driver at fault in the event an accident occurs because almost all autonomous vehicle systems (including Tesla’s Autopilot) require a human operator to be ready to take over at all times when operating their vehicle in autonomous mode.
But FSD is different. It includes features such as summoning that allow the vehicle to operate without a driver on standby. Furthermore, as a software add-on, it’s not even tracked in the vehicle identification number you give your insurance. This means there’s no real answer as to who, exactly, is responsible if your Tesla runs somebody over valeting itself.
Of course, you can always buy insurance directly from Tesla. According to this website , the company offers “autonomous liability” coverage. But, the point is: there’s no current regulations requiring people who own cars with autonomous capabilities to differentiate between hands-on systems and beta tests for hands-off ones.
The problem
The reason FSD is stuck in beta is because it’s simply not ready for the mainstream. Legally speaking, it would likely be catastrophic for Tesla to release FSD to all its vehicle owners and assume liability for millions of self-driving cars. There is absolutely no reason to believe FSD, in its current iteration, is ready for safe mainstream use.
In fact, Tesla is very clear on its own website that FSD is not a finished product:
FSD is a hodgepodge of really great ideas executed well. It’s a modern marvel of technology and, if you ask this humble tech writer, Teslas are the best cars on the planet. But they are not fully self driving no matter what Elon Musk calls the software powering their limited autonomous features.
But, no matter how stupid the product is named, the fact it doesn’t work right isn’t really Tesla’s fault. If the roads were kept in perfect shape and all the cars on them were driven by Tesla’s FSD/Autopilot system, it’s almost a certainty that millions of lives would be saved. Unfortunately, unless Musk plans on giving every eligible driver a free Tesla, most of us aren’t going to have them.
And FSD isn’t ready to handle the unpredictable nature of pedestrians, human drivers, crappier cars with worse safety standards falling apart on the roads, pot holes, mattresses and other trash in the middle of the road, logs falling off of big rigs, and myriad other situations that aren’t easily understood by a computer interpreting data from a bunch of cameras in real time.
The solution?
You shouldn’t be surprised to know there isn’t one. That is to say, we’re already doing our best. Most carmakers are heavily invested in driverless cars and it’s pretty safe to say the majority of academics and pundits all agree that letting robots drive cars will eventually be much safer than putting humans behind the wheel.
The technology isn’t there for Tesla’s inside out approach involving on-board hardware and cameras. At the end of the day, we’re still talking about image recognition technology: something that can be fooled by a cloud , a hand-written note , or just about anything the algorithm isn’t expecting.
And other approaches, such as Waymo’s robotaxi tests in Arizona , rely on a very specific set of circumstances to function properly. A million safe miles picking up and dropping off pedestrians between designated travel points, during specific times of the day, is not the same thing as logging time on the wildly unpredictable streets of New York, Berlin, Hong Kong, or anywhere else the computer hasn’t trained on.
The reality
Self-driving cars are already here. When you look at their capabilities piecemeal, they’re incredibly useful. Lane-switching, cruise control, and automated obstacle avoidance and braking are all quality of life upgrades for drivers and, in some cases, literal life savers.
But there’s no such thing as a consumer-marketable, mainstream self-driving car because those don’t exist outside of prototypes and beta trials. And that’s because, in reality, we need infrastructure and policies to support autonomous vehicles.
In the US, for example, there’s no consensus between federal, state, and local governments when it comes to driverless cars. One city might allow any kind of system, others may only allow testing for the purpose of building vehicles capable of connecting a city’s smart grid, and still others may have no policy or ban their use outright. It’s not just about creating a car that can park itself or enter and exit a freeway without crashing.
That’s why most experts – who aren’t currently marketing a vehicle as self-driving – tend to agree we’re probably a decade or more away from an automaker selling an unrestricted consumer production model vehicle without a steering wheel.
We’ll likely see robotaxi ventures such as Waymo’s expand to more cities in the meantime, but don’t expect Tesla’s Full Self Driving to come out of beta any time soon.
Here’s why the US continues to beat China in the AI race
The global AI race was supposed to be a sprint. Back in 2017 when driverless cars and domestic robots were thought to be just around the corner, the promise of deep learning made it seem like we were mere months away from living in an AI-powered utopia.
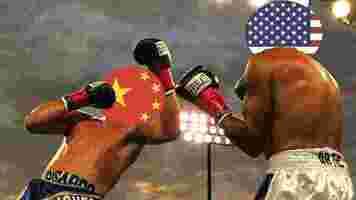
As it turns out, the global AI race is more of a marathon. And the US has a huge lead that’ll be difficult to overcome for any country, but especially China.
The setup
It was easy to believe China would pull ahead a few years ago. US big tech companies such as Microsoft and Apple had always co-existed with eastern outfits. But, once deep learning exploded in 2014, many experts believed China would use its government influence to direct the flow of research in ways the EU and US’ respective leaders simply couldn’t.
And, for a while, it looked like that was going to be enough to propel the PRC to the top of the global AI leaderboards.
In the west, a lion’s share of AI research ends up patented by businesses who keep their algorithms in a walled-garden. But in the east things are different.
Per an article in the Harvard Business Review :
China’s big problem
The biggest problem China has when it comes to AI is a lack of innovation. Consumer demand is at an all-time high for deep learning technologies in China, but this social trend isn’t translating into breakthroughs.
In essence, China is still playing catch up. The Chinese government may be pouring more money into research and producing more of it, but US tech companies are raising and spending more on research outside of academia.
The US government still spends more on defense AI than China, and US businesses spend more money on cutting-edge research than Chinese companies do.
Simply put, the biggest technology companies in the US can afford to invest in breakthrough research even when such research leads nowhere. The profit margins are much leaner at most Chinese firms so the incentive is typically on producing a profit .
Unfortunately for China, much of its AI position is rooted in developing Chinese-language versions of language recognition software and creating surveillance technology – neither of those are very marketable outside of places where Chinese is spoken or where privacy laws exist.
What it all means
Deep learning might not be the best path forward for artificial intelligence technologies. This is great news for big tech companies in the US. But it’s bad news for China.
In the US, where most of the AI breakthroughs tend to come from big tech companies with large enough coffers to afford supercomputers and high enough salaries to lure away academia’s brightest, scientists won’t miss a beat if we transition away from deep learning.
But China’s heavily-saturated market likely won’t extend beyond its own bubble, much less the deep learning bubble that could pop and leave AI-only companies behind. There’s a reason why there’s only one Chinese firm among the top five richest technology companies in the world.
It’ll be tough for academia in China to keep up with big tech in the US no matter how much data it can generate or acquire.
We’re more likely to see these kinds of catch-up cycles end in cooling-off cycles when heavy government investment doesn’t pay off. China could be headed for an AI winter.
COVID-19 made your data set worthless. Now what?
The COVID-19 pandemic has perplexed data scientists and creators of machine learning tools as the sudden and major change in consumer behavior has made predictions based on historical data nearly useless. There is also very little point in trying to train new prediction models during the crisis, as one simply cannot predict chaos. While these challenges could shake our perception of what artificial intelligence really is (and is not), they might also foster the development of tools that could automatically adjust.
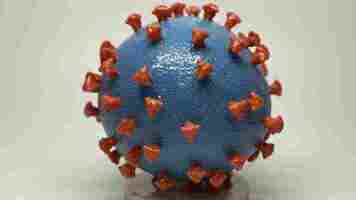
When it comes to predicting demand or consumer behavior, there is nothing in the historical data that resembles what we see now. Thus, a model based purely on historical data will try to reproduce “what is normal” and is likely to give inaccurate predictions.
Let me give you a simple analogy of the problem that data scientists and machine learning professionals are now experiencing. If you want to predict how long it is going to take to drive from A to B in London next Thursday at 18:00, you can ask a model that looks at historical driving times, and possibly at various scales. For instance, the model might look at the average speed on any day at around 18:00. It might also look at the average speed on a Thursday versus other days in the week, and at the month of April versus other months. The same reasoning can be extended to other time scales as one year, ten years, or whatever is relevant for the quantity you are trying to predict. This will help predict the expected driving time under “normal” conditions. However, if there is major disruption on that particular day, like a football game or a big concert, your travelling time might be significantly affected. That is how we see the current crisis in comparison with normal times.
Perhaps unsurprisingly, many AI and machine learning tools deployed across various businesses – from transport to retail, professional services and the likes – are currently struggling in trying to cope with massive changes in the behavior of both users and the environment. Clearly, one can try making prediction algorithms focus on smaller parts of data. However, it is also pretty obvious that one cannot expect “normal” outcomes and the same quality of predictions as before.
What to do?
There is some good news for data scientists and the likes though. Generally, data science solutions are built on historical data, but current, “extraordinary” data should come in when continually assessing the performance of those existing solutions. If performance starts to drop off consistently, then that can be an indication that the rules have changed.
This performance monitoring is independent of predictive systems for now – it tells us how things are doing, but will not change anything. However, I believe that we are now seeing a major push towards systems that could adjust automatically to the new rules. This is something we can call “adaptive goal-directed behaviour”, which is how we define AI at Satalia. If we can make a system adaptive, then it is going to adjust itself based on that current data when it recognizes performance dropping off. We have aspirations to do this, but we are not there just yet. In the short run, however, we can do the following:
Do not try to train a brand new model from Day 1 of the crisis, it is pointless. You cannot predict chaos;
Gather more data points and try to understand/analyze, how the model is affected by the situation;
If you have data from a previous crisis with similar characteristics, train a model on that data and test it offline to see if it works better;
Make sure your training data is always up to date. Every day, the new day goes into the data and the oldest day goes out. Like a sliding window. The model will then gradually adjust itself;
Shrink the timeline of your dataset as much as possible without affecting your metrics. If you have a very long dataset, it will take too long for it to adjust to the new reality; and
Manage client expectations. Make it clear that noise is making things very hard to predict. Computing KPIs during this time is next to impossible.
Clearly, building a model that is able to respond to extreme events may incur significant extra costs, and perhaps it is not always worth the effort. However, should you decide to build a model that is able to respond to extreme events, then they should be considered during development/training. In this case, make sure to capture the long- and short-term history of your data when training the model. Assigning different weights on long- and short-term information will enable you to adapt more sensibly to extreme changes.
In the long run, though, this crisis reminded us that there are events so complex even we humans still struggle to understand, let alone predictive systems we have built to systematize our understanding in normal times. Even us humans need to adapt to this “new normal” by updating our own internal parameters to help us better forecast how long it will take to do the weekly shop or choosing a new optimal path when walking down the street. This adaptability is natural for us humans and it is a feature we should be constantly trying to impart on our new silicon work colleagues. Ultimately, we need to recognize that an AI solution can never be seen as a finished product in the ever-changing and uncertain world in which we live. How we enable AI systems to adapt as efficiently as we do – in terms of the number of data points – is very much an open question whose answer will define how much our technology will be able to be of help during the extremely volatile times that might be ahead of us.
I thank my colleagues Alex Lilburn, Ted Lappas, Alistair Ferag, Sinem Polat, Jonas De Beukelaer, Roberto Anzaldua, Yohann Pitrey and Rūta Palionienė for providing insights and helping me to prepare this article.