WTF is Science Corp? Neuralink co-founder creates secretive brain-hacking company
Did you know Neural is taking the stage this fall ? Together with an amazing line-up of experts, we will explore the future of AI during TNW Conference 2021. Secure your online ticket now !
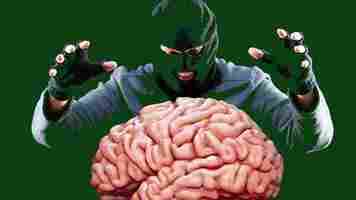
It’s tough out there for a supervillain. All the best talent goes to Google and Amazon, Elon Musk‘s hogging the spotlight, and everyone’s focused on modern evil stuff like cryptocurrency and NFTs – nobody cares about the classics anymore.
Well, Science Corp is here to change that with some good old-fashioned brain hacking.
Probably. Maybe. Who knows? Honest question: WTF is Science Corp ?
Futurism’s Simon Spichak broke the news about the company earlier this week with the careful-not-to-spit-out-your-coffee-when-you-read-the-rest-of-this-sentence-news that Science Corp had already raised a massive $48 million funding (what?!?).
Here’s what we know so far:
And that’s about it. We reached out to the people associated with the company we could identify, but haven’t received a response from anyone yet.
[Related: A brief history of Mt. Gox, the $3B Bitcoin tragedy that just won’t end ]
Despite the fact the company‘s being secretive, we can still glean a few potential insights.
Firstly, as Spichak points out in their article:
It’s possible this all adds up to a new non-invasive brain-computer-interface that sends light through the eyeball to interact with the brain.
It’s more likely, however, that the whole eyeball-science part of the endeavor is linked in with an invasive (read: drilling holes in your head) device similar to Neuralink’s… but with whatever upgrades Science Corp’s new ideas would add to the mix.
But here’s the thing: unless this company is taking Neuralink’s tech and running with it, we can’t expect a serious look at what it’s trying to accomplish for a year or more.
In fact, the Futurism article mentions that it was never really clear if Hodak left Neuralink in good graces or was fired due to “moving too slow on clinical trials.”
So we’re guessing that speed isn’t the name of the game here. But… what is?
It’s understandable when a new company doesn’t want to alert the general public to its presence until it has a full team in place. But when said company has already raised a gobsmacking $48 million and plans to do… something … with people’s brains, it raises some eyebrows.
The next question we have to ask is: who or what is overseeing these brain-hacking startups? Is there a government medical board or third-party science advisory committee making sure nobody’s trying to perform brain transplants with pigs and dogs or something?
Read Futurism’s whole article here .
H/t: Jon Christian, Simon Spichak, Futurism
4 ways AI is unlocking the mysteries of the universe
Astronomy is all about data . The universe is getting bigger and so too is the amount of information we have about it. But some of the biggest challenges of the next generation of astronomy lie in just how we’re going to study all the data we’re collecting.
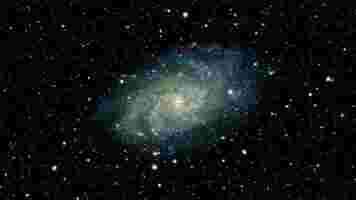
To take on these challenges, astronomers are turning to machine learning and artificial intelligence (AI) to build new tools to rapidly search for the next big breakthroughs. Here are four ways AI is helping astronomers.
1. Planet hunting
There are a few ways to find a planet, but the most successful has been by studying transits . When an exoplanet passes in front of its parent star, it blocks some of the light we can see.
By observing many orbits of an exoplanet, astronomers build a picture of the dips in the light, which they can use to identify the planet’s properties – such as its mass, size and distance from its star. Nasa’s Kepler space telescope employed this technique to great success by watching thousands of stars at once, keeping an eye out for the telltale dips caused by planets.
Humans are pretty good at seeing these dips, but it’s a skill that takes time to develop. With more missions devoted to finding new exoplanets, such as Nasa’s ( Transiting Exoplanet Survey Satellite ), humans just can’t keep up. This is where AI comes in.
Time-series analysis techniques – which analyse data as a sequential sequence with time – have been combined with a type of AI to successfully identify the signals of exoplanets with up to 96% accuracy .
2. Gravitational waves
Time-series models aren’t just great for finding exoplanets, they are also perfect for finding the signals of the most catastrophic events in the universe – mergers between black holes and neutron stars.
When these incredibly dense bodies fall inwards, they send out ripples in space-time that can be detected by measuring faint signals here on Earth. Gravitational wave detector collaborations Ligo and Virgo have identified the signals of dozens of these events, all with the help of machine learning .
By training models on simulated data of black hole mergers, the teams at Ligo and Virgo can identify potential events within moments of them happening and send out alerts to astronomers around the world to turn their telescopes in the right direction.
3. The changing sky
When the Vera Rubin Observatory , currently being built in Chile, comes online, it will survey the entire night sky every night – collecting over 80 terabytes of images in one go – to see how the stars and galaxies in the universe vary with time. One terabyte is 8,000,000,000,000 bits.
Over the course of the planned operations, the Legacy Survey of Space and Time being undertaken by Rubin will collect and process hundreds of petabytes of data. To put it in context, 100 petabytes is about the space it takes to store every photo on Facebook , or about 700 years of full high-definition video.
You won’t be able to just log onto the servers and download that data, and even if you did, you wouldn’t be able to find what you’re looking for.
Machine learning techniques will be used to search these next-generation surveys and highlight the important data. For example, one algorithm might be searching the images for rare events such as supernovae – dramatic explosions at the end of a star’s life – and another might be on the lookout for quasars. By training computers to recognise the signals of particular astronomical phenomena, the team will be able to get the right data to the right people.
4. Gravitational lenses
As we collect more and more data on the universe, we sometimes even have to curate and throw away data that isn’t useful. So how can we find the rarest objects in these swathes of data?
One celestial phenomenon that excites many astronomers is strong gravitational lenses . This is what happens when two galaxies line up along our line of sight and the closest galaxy’s gravity acts as a lens and magnifies the more distant object, creating rings, crosses and double images.
Finding these lenses is like finding a needle in a haystack – a haystack the size of the observable universe. It’s a search that’s only going to get harder as we collect more and more images of galaxies.
In 2018, astronomers from around the world took part in the Strong Gravitational Lens Finding Challenge where they competed to see who could make the best algorithm for finding these lenses automatically.
The winner of this challenge used a model called a convolutional neural network, which learns to break down images using different filters until it can classify them as containing a lens or not. Surprisingly, these models were even better than people, finding subtle differences in the images that we humans have trouble noticing.
Over the next decade, using new instruments like the Vera Rubin Observatory, astronomers will collect petabytes of data, that’s thousands of terabytes. As we peer deeper into the universe, astronomers’ research will increasingly rely on machine-learning techniques.
This article by Ashley Spindler , Research Fellow, Astrophysics, University of Hertfordshire , is republished from The Conversation under a Creative Commons license. Read the original article .
This virtual training suite trains a robotic arm to move objects in complex scenarios
The Allen Institute for AI just announced a new framework called ManipulaTHOR that helps in creating various real-life scenarios to train a robot arm in manipulating objects.
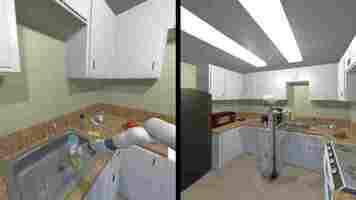
This new testing suite — part of the AI2-THOR update — has more than 100 physics-enabled physics-enabled rooms that have complex environments and obstructions that might get in the way of a robot arm while interacting with objects. ManipulaTHOR will enable faster training in more complex environments without needing to build real robots.
The new framework update allows these simulated robots to move in these rooms like humans and perform tasks such as navigating a kitchen, opening a fridge, or popping open a can of soda. Plus, through these features, robots are able to move objects in a room swiftly and accurately — despite many hindrances.
A lot of robots are trained to move in a very specific manner, and they find it difficult to overcome obstacles, making them unsuitable for a lot of real-life scenarios. This framework provides a way to solve such problems first in a virtual world, so some of those concepts could be applied to physical robots later.
The team has modeled this virtual arm based on the design of the Kinova Gen3 Modular Robotic Arm specification — a real-life robot. The platform allows the arm to move in six degrees of freedom. Plus, there are virtual sensors such as egocentric RGB-D images and touch sensors to better gauge the room and objects.
Roozbeh Mottaghi , research manager at AI2, said that this framework allows researchers to simulate a ton of scenarios safely and quickly:
You can learn more about the ManipluaTHOR framework here .